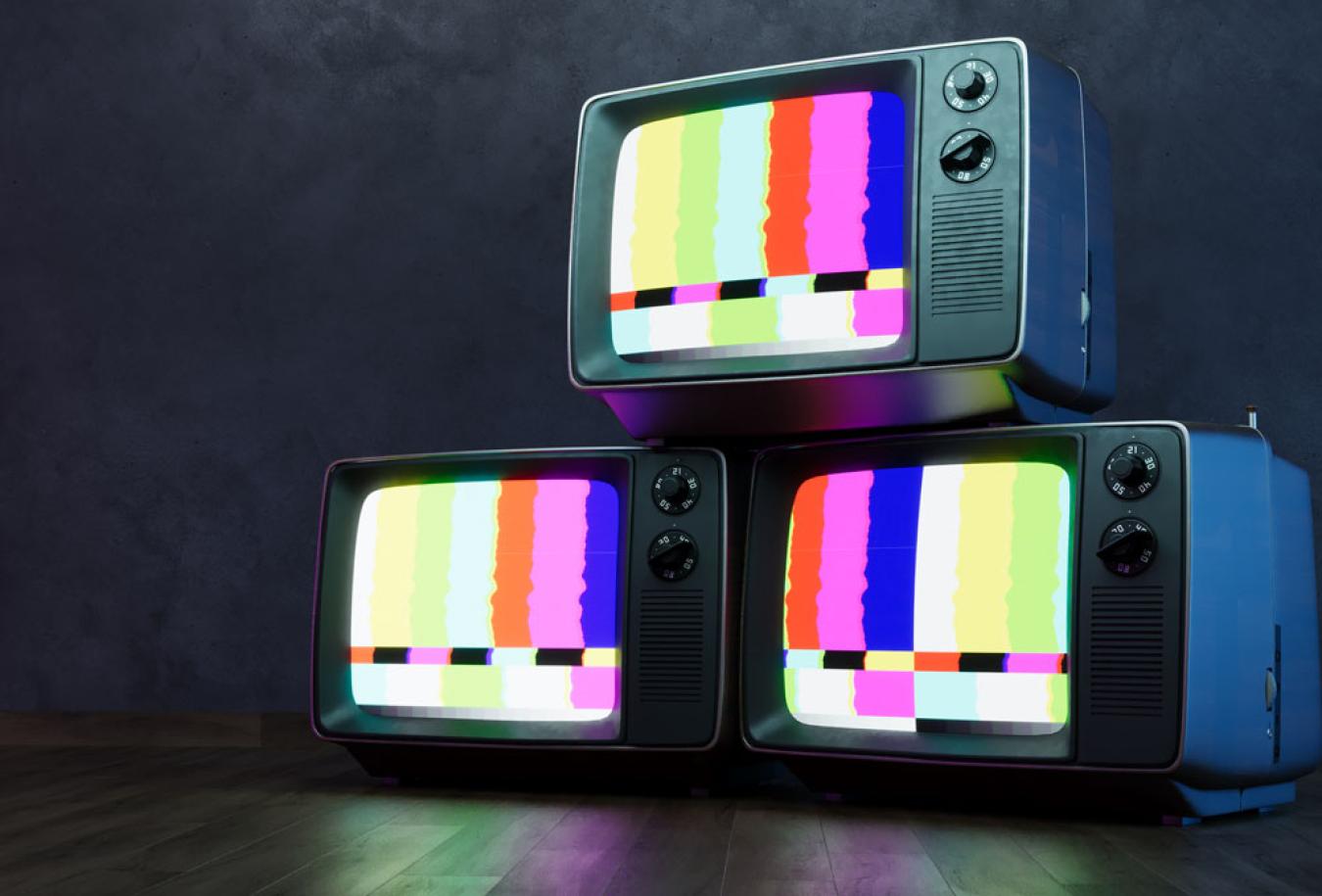
Panel Members: Kathy Reid, Peter-Lucas Jones (hosted by Keir Winesmith)
From voice-activated devices to speech-to-text tools, voice recognition technology has grown in the last 15 years to become an indispensable part of modern living. As we tread alongside the path of its development, we pause to ask: who gets left behind? Kathy Reid (ANU), Peter-Lucas Jones (Te Hiku Media) and Keir Winesmith (NFSA) discuss bias in voice data, weaknesses in certain demographics and leading the movement to take ownership of language technologies away from American giants.
Technology, language, history and creativity converged in Canberra for four days as cultural leaders gather for the world's first in-depth exploration of the opportunities and challenges of AI for the cultural sector.
Want to learn more about this event?
Visit the Fantastic Futures 2024 Hub
This transcript was generated by NFSA Bowerbird and may contain errors.
In lieu of long introductions for our two key speakers, because they're on the website and you can find them on the internet, I'm going to do a different sort of introduction, a really quick one, so we can really get into the storytelling. When we started talking about the mass transcription that was sort of necessary after we've moved into mass digitisation and sort of to enable mass discovery at the NFSA, I asked around, who should I talk to? Who's doing work in the speech-to-text world, in the transcription world, in the kind of entity world? Who's doing it ethically, doing it successfully, and doing it in a kind of Australia or New Zealand context? And two names keep coming up from the people I asked. And those two names were Kathy Reid at ANU and Peter Lucas Jones at Te Hiku Media. And so I went and spoke to those two people, and I've learned a lot from them. And so for the next 45 minutes to an hour, you all get to learn a lot from them as well. So please join me in welcoming them to the stage. Oh, did I say who I am? No? Sorry, whoops. So my name is Keir Winesmith. I'm the Chief Digital Officer here at the National Film and Sound Archive, and convener of today's and tomorrow's conference. Kathy, I'm gonna start with you. Sure. And we're gonna start at the beginning, and then it's you, Peter, we're also gonna start at the beginning. Where did speech, sorry, speech recognition technology first emerge, and what problem was it originally designed to solve? What a brilliant question. So speech recognition technology has had, we think of it as a very new technology, but it's something that goes back centuries, if not decades. So there's a really early linguist who worked with Indic languages called Parmini, and his original work looked at how speech patterns in those Indic languages and he was one of the first people to create something called a phoneme which is a basic building block of sound and we still use some of his original work today. So that's going back to I think the 4th to 6th century AD. So we think of speech recognition as a very, very new technology but it has a history like many things do. that go back centuries. And really, speech recognition, the advances in speech recognition, really came about as a military technology. So if we cast our minds back to the Cold War of the late 1940s, 1950s, into the 1960s, there was a real desire to see, or real desire to be able to understand the language of people who we were perhaps a little bit are a little bit afraid of and wanting to make sense of what they were saying and I'm sure they wanted to make sense of what we were saying about them as well. So speech recognition has a history as a military technology and I think the really important part of this story is the turn to data, the datafication of speech. So when we think about speech we think about audio and we think about the sounds that we make. But in, you know, beginning in the 1960s and through the 1970s, speech recognition became a practice of statistics, a practice of data, and speech was really data-fied. And so now if I fast forward to today, we have speech recognition technologies everywhere. If you're on Zoom, you get transcribed. If you're on YouTube, you get transcribed. You speak to your phone. I won't say any wake words and wake up your phones. It's OK. So we have that in many languages. So we've got about 7,000 languages that are spoken in the world today. In English, I can talk to my microwave as long as I use an American accent. But for many of the world's spoken languages, many of the world's 7,000 spoken languages, we don't have those technologies. We're not afforded the benefits of those technologies. And as William Gibson once said, the future is already here. It's just not distributed. And the quote that I would sort of take that to the future's already here but the futures we're creating are not evenly distributed because the data that we have is not evenly distributed. Wow, thank you. So that took us from AD-ish to today-ish. Peter, what are the problems with this approach, especially for people outside America? I guess the further you go from Idaho, the less accurate these data-fied speech recognition tools are. What are the problems for people here in New Zealand, especially for people who don't speak English as their first language? Oh, kia ora. First of all, I would like to acknowledge the Nanawa people, and of course, that beautiful welcome that we had from Aunty Violet, because it's moments like those that enable us to remember. And I think remembering how we got to where we are, it highlights things that are sometimes forgotten. And just picking up on what you said, Kathy, I would add that speech recognition, certainly in Maori context, I'm from New Zealand, and I'm from Te Aupouri, which is one of the far northern tribes. But what I would say is speech recognition has also been used as a tool of invasion. And whilst we think about what invasion means in the past, and I think of a proverb and it's, e hoki whakamuri ki a ngā whakamua. And what that means is to really take on board what's occurred in the past as we design what might be a preferred future. And I say that because language is the key to culture. And often when we think about these types of topics, we're applying a cultural perspective that is deeply rooted in Western history, in Western construct. And I think when we do that, we actually miss an opportunity to grow. And your question about, essentially, for me it's about who's forgotten? Everybody else is forgotten. And when we think about what that means, language being the keys to culture, culture is the home of vast bodies of knowledge. And for languages like Te Reo Māori that were not originally written languages, our experience with orthography is often associated with white assimilation. And so the orthography of our language, for example, has, I guess it's been through different phases of development. And we share some features of orthography with other Pacific languages, I guess, that were being Christianized at the same time. The reason I mention this is because speech-to-text technology very much works well when you have a particular sound. And you mentioned that earlier, accent. But it is possible to have reasonably good pronunciation and still speak with an accent, as we all know. I think to answer your question, when we think about those 7,000 languages that were mentioned by Kathy, many of them will be a memory in 2015. And when we think about what happens when a language dies, we remind ourselves that as indigenous people, every time we lose a word, we lose part of our culture. And so intergenerational transmission of language and culture through technology will be a powerful learning tool and acquisition tool for our mokopuna, for our grandchildren. And I think that's an important feature of the work that I'm involved in. But it's also something that people need to be aware of as they are contributing to work that could well impact those communities that may well risk losing their language if we don't have the level of, I guess, understanding, but also support, because it's important to be able to understand how to enable AI for good. And I think that's an important part of this process. There's a lot in there. I think some of our American and European audiences will be surprised to hear our children talking to their devices in American accents because they work better if you do that. I think that's something you probably don't realise when you come to kind of, let's say, low resource parts of the world. Can we stay on accents for a minute? I know you've done a huge amount of work, especially through your PhD, on accents. Take us through from the most accurate to the least accurate and kind of what the impacts of that kind of density and identification is, Kathy. Sure thing. So just to provide a little bit of context with my PhD research, as I was sort of doing my PhD research, which was looking at the data that goes into speech recognition and the voice data that goes into speech recognition, I'm actually going to start not with accents, but with age and with gender. Sorry, it's my prerogative. I get to take a departure point. So, I work very closely with the team at Mozilla Common Voice. Some of you will be familiar with Common Voice. It's the world's largest open data set of speech data. I think there's currently 113 languages in Common Voice. And Common Voice also started to collect demographic data very much opt-in of its speakers. And earlier in my PhD, I did some data visualization work with Common Voice looking at, you know, why do we have so many biases in speech recognition? And I thought that data was one of the key things there. And you'll see here that These are the top eight languages in common voice. They're the languages that we have the most data for. One of the things you'll note there that we have more language, more speech data in common voice for Catalan, a language spoken in northern Spain, than we do for English. That's largely as a result of the Catalan independence movement, and I think there's a lesson there. But we'll see that we have very particular amounts of data for speakers of different ages. So Catalan is the only language in common voice that has a very good spread of age data. And what this means is that when we use common voice data to create speech technology and to create speech recognition models, they're not going to work well for people, for speakers who we don't have data for. So this is the age spread, and you can see that we don't have age data for a lot of that data. But the data that we do have age metadata for is basically for very young people in their teens, 20s, 30s, 40s. When you start to get into your 50s, 60s, 70s, we don't have a lot of that speech data. So I think that's something to be very mindful of. And Vanessa, I might get you to go to the gender slide as well. Vanessa's doing a brilliant job of DJing all the slides for us today. We see very similar issues in gender splits as well. So we have much more data for male speakers, people who identify as male, than we do for people who identify as female. I recognize that we have much more than binary gender as well. This has huge impacts on how well speech technologies work for people of different cohorts. Now I'm going to turn my attention to accents. Looking at all of this, I started to think about accents. an accented speech. And one of the things that I did with Common Voice was look at what are the accent representations that we have within that data set for English speakers. And one of the data analyses I did, we can't see it very well on the slide, but you'll see that the very big circle in the middle there is speech data in common voice that is tagged as having a United States English accent. And all of those other tiny little dots are the accents that are represented in common voice. And one of the pieces of work that I did in my PhD was some manual taxonomic classification of all of those accents in common voice. And then what I was able to do, once we have all of this taxonomic data, I threw the entire common voice corpus of English, which is about 2 million records, I threw that at the Whisper speech recognition engine to see what it would tell us about how well Whisper worked for some of those accents. I'm going to get a show of hands in the audience. I know we have at least one person who speaks with a Scottish accent. Do we have any other Scots folk in the house? A few? Fawlty. I'm very sorry. Whisper does absolutely appallingly on Scottish English. Absolutely, appallingly. And if any of you have seen the YouTube clip with the people in the elevator that's voice activated trying to say 11 to go up in the elevator, I thought that was just a bit of a joke until I threw Common Voice at Whisper. And you can see here on the left there that Scottish English performs absolutely the worst. It's not the worst accent, but it's one of the worst accents for the Great Britain region in common voice. So if you speak Northern Irish, Whisper actually does a very good job of understanding what you're saying, but if you speak Scots, or if you're from the North West country in Lancashire, it's not going to understand you particularly well at all. So, yes, that's a little bit of my PhD research and why whisper doesn't work very well on accented speech. And an apology for all the Scottish speakers in the audience. That's fantastic. And the data doesn't lie. The data reflects the biases that are driven into the data. And some of the work, Peter, you've been doing with Teku Media, in particular with Tereo, is because of that bias in the raw data. How have you been addressing that with your community? Well, with our community, we've really focused on community participation. And we often hear about crowdsourcing. And I think that when we look at things from an indigenous perspective, and we take on board the difference of what crowdsourcing means versus what community participation means, it really draws our attention to capability development and capacity development that can occur alongside wanting to achieve results in this area. And for us it's really been about understanding that the sound the sound that might be an accent, the sound that might be a representation of a tribal variation of the language or a dialect, is very much connected to the people and the place that are associated with an identity. And that identity is often something that has been diminished through what many of our grandparents and great grandparents or even our parents went through when they had the language beaten out of them. And so it represents a moment to reconnect. And so for us, it's been very much about understanding the data. What we're dealing with in our speech technology development is the fact that we're dealing with a limited amount of data. We hear people talk about large language models, and those are all extremely relevant. However, our data problem is the fact that we come from a minority language group that has a diminished number of speakers. So what we've focused on is meticulously tagging and labelling all those features, those phonetical features that you've already mentioned, that are representations of accent. But when we hear people speak about accent, we're often taken to a place where accent only occurs when someone speaks English. But as a speaker of another language, I can say that accent also occurs when learners of a language, for example, learners of Te Reo Māori, speak that language. And you can hear that. And I think it's important to mention how important re-indigenizing the sound of our language is, because in so we recognize the influence of English on pronunciation, and when we talk about pronunciation, we're talking about speaking native sound into our future. and we treat our archives not only as a repository of knowledge, but they are a very, very well curated data set that we have meticulously tagged and labeled to ensure that the representation of the language, for example, in our synthetic voice development, is an accurate or near-to-accurate or authentic representation of our language whilst it is synthetic. We've also taken into account that code-switching for indigenous language speakers is a reality, and we code-switch between Māori and English. depending on the language proficiency of the speaker or the person that you're speaking with, or preferred words, for example. And I think that's an important thing for us to recognize, because minority languages are often dealing with new words for new nouns, for example. You know, do we call it an ATM? Or do we call it something else? And it's up to the speaker, of course. But what I'm saying is speech recognition and speech technology in our own experience has been something that we've developed for a lot of reasons. because we want to be able to search and find our own knowledge in our own data sets as well. And I think that speaks to data sovereignty. And we can't really have these sorts of conversations without thinking about intellectual property rights and the need to uplift the communities that we belong to at the same time as developing these technologies. because we come from communities that have largely been the recipients of treatments or regimes or government decisions that have left those communities not only landless, are resourceless, and as we sort of grow into the modern context, we can't develop these things without taking into account the other issues that surround our health and well-being. And so I think it's important that we take into account the context, the cultural context, and the need for economic development to be a focus of this work while we're doing it. When we first spoke, Peter Lucas, it was before Whisper, it was before ChatGPT, it was before COVID, in fact. And I remember chatting with you on the phone from Australia and you were saying, well, we have this incredible resource around us. and you were in this really, like you described it as, like a really kind of down at home office, like faded carpets, like plasterboard wall, and I was looking over your shoulder, and you were pointing around to say, look at all these incredible resources we've got, and I didn't know what you meant, and you walked the camera on the laptop into the studio, and the resource was your community radio station, and the resource was years of native speakers and the resource was a committed community who wanted to tag their culture through language so that you could build something to compete with what was coming out of America. You could build something from within the community. The resource was that commitment to language. What kind of head start did that give you in this work? Well, it just reminded us the very people that beat the language out of our parents could very well be the ones selling our language back to our grandchildren as a service. Now that raised concerns for us. That was problematic. We come from a remnant native speaker community and the reason that we have a remnant native speaker community and the language decline and the cultural decline in our specific community or tribal group is not as severe as it is in other tribal areas. is because many people believe that our land and our resources, natural resources, were not economically as valuable as the land and resources of the other indigenous people that were a little bit further away from us. And so our context provided us with a little bit more time for intergenerational transmission of language and culture to occur. And so in that we have a resource, a living resource of speakers and native speakers, second language learners, and we have worked closely with our listeners. We are one of 21 iwi radio stations. And those 21 iwi radio stations all have a different context, a different context in which they are conducting their efforts for language revitalization and cultural revival to occur. Ours has always been working with our community, because our data is stored in song, our data is stored in dance, our data is stored in the landscape, our data is very much stored and connected with the natural environment and our stories about, for example, why you take the bark from the tree. that the sun shines on, and not the bark from where the shadow falls. Why do we talk to our elders about these things in our language? Because we have an opportunity to. And so we record all that information, but whilst recording that information, we have the opportunity to record it in our language. And so in recording, whatever our people want to talk about and speak about, we're not only recording a repository of knowledge, we're recording natural language. And that language that we have been gathering for more than 30 years has been the backbone of the work that we do. We recognise, however, that working with our grandparents, and I do want to recognise Honiana, because Honiana is here and she'll be able to talk a lot more about, for example, our cassette tapes. When we started digitising our cassettes, It posed a new problem, because all our information was safe when it was in that box in the room. There was a big movement to digitize everything. Digitize, digitize, digitize. Because it was going in the cloud. And where was the cloud? Oh, we didn't know. Nobody really knew that it was just a room on someone else's land, using someone else's natural resources, turning into a climate catastrophe on the land of another indigenous people. So it made us think. It made us think about how do we bring services into our community and provide our own services? How do we think about indigenous climate justice alongside the fact that we wanted to lead the development of automatic speech recognition for our own language working with our community? But in closing, because I could go on about this for a little while, What I would say is that what we've created is something that our neighboring tribal groups could benefit from, our sister languages could benefit from, and the development of a multilingual Pacific language model is not out of sight. And I think collaboration for indigenous-led solutions is something that needs to be supported. Because when we think about self-determination, I'm thinking about how do we enable capability and capacity development to happen alongside these amazing things that are happening. Thank you, Peter Lucas. When we have talked in the past, and I think it's coming up later in today and probably tomorrow and probably forever, this language of data sovereignty, in particular for First Nations, I think the word sovereignty is a complicated word in Australia for all the reasons that Aunty Violet spoke about just before. So we could talk about it as agency or capacity, as you've been using those sorts of words. That's on the outside as it goes forward. How it comes in is really critical. And a lot of your kind of research, Kathy, has been about what are those data sources for things like common voice, which is, you know, interrogable. But for a lot of the tools that animate the products that we use every day, we can't see inside them. What would we see if we could see inside them? What a great question. If only someone had done some research on this. One of the things that really irked me a little bit about Whisper, and before I go into what irked me, I just want to draw some broader context about Whisper. So Whisper was released in 2022 and for those who don't spend their evening studying speech recognition models, Whisper is an automatic speech recognition model that is freely available. I'm not going to say open source because it's very much not open source. It's freely available and it's becoming, it has become increasingly dominant in terms of which speech recognition models are used, particularly in areas, because it's freely available, particularly in areas that need to transcribe huge volumes of content, because the way that business models for other forms of automatic speech recognition in the cloud work, you basically pay per minute of transcription. And so, Whisper has started to displace those paid models. And it's really reached a position of, it's become ascendant, it's become a dominant model in the space within 12 to 18 months of inception. So Whisper was trained on 680,000, or version two of Whisper was trained on 680,000 hours of speech data. Version three was trained on, I think, four million from memory. I'd have to check the paper. But if you ask the question, where did that 680,000 hours of speech data come from? If you read the paper, it has a tiny paragraph that says, we took video transcripts from the internet. which is an incredibly descriptive part of methodology. If you look very closely at how Whisper does transcription and at some of the hallucinations that Whisper does, which is some of the other research I've done, it hallucinates things like, please like and subscribe, or don't forget to click the bell. Now, I can't imagine where those transcripts might have come from, can you? I have a couple of ideas. So there's all sorts of questions there about, if you have recorded a video for a particularly very well-known video website, and that has been transcribed, did you have any idea that that data might end up in a model that OpenAI is now selling? So you can buy Whisper as a service from OpenAI. And whisper is also being used in multimodal models. So a multimodal model in artificial intelligence is a model that does text, image, video, speech, multiple modes of communication. So, for those of you who use the new models from OpenAI, Sora, the O models, a lot of what Whisper did with speech recognition is baked into those models, into those speech-to-speech models. And so, when data is created, it has this incredible sort of downstream history, if you created a video, put it on a particular video website that will remain nameless, it has been scooped up, harnessed, data-fied, turned into tokens, trained with the GPU, it's in Whisper, it's in Sora, and it will probably be in the metaverse in some form or another. So we don't have very good control over what data goes into speech recognition. We don't have very good provenance. And the people who are making these models don't tell us what data is in there either. And it's up to researchers to sort of reverse engineer what might be in them. Cautionary tale. This will come up, I think. There's a number of whisper-centric talks later today, including some from the NFSA. Peter Lucas, given everything we've just described, where things come from and then where they go, one of the things that you and your team do so well, I think, is ground the work, even the pronunciation work, in country, in place, and in community. There are other institutions that have these rich sources of data and many of those institutions are represented today. What advice, you know, someone who's been doing this from before it was cool, let's be honest, what advice would you have for cultural institutions and universities and libraries who have these large collections of often you know, extracted stories or extracted culture, but often, you know, donated and given culture, what should they be thinking about as they realize that, as Patrick said earlier, you know, these tools are an unlock for parts of our collections that even cultural workers in our institutions don't know what they have. What should they be considering? What should they be thinking about? What have you learned in the sort of almost 10 years you've been doing this work that you can share? I'd start off by just saying that Benchmarking is a really important thing, particularly for marginalized languages. And big tech is eroding the quality that we hold up in such high esteem, the quality of language recognition. And I wanna just draw our attention, I'm gonna get to your question. I want to draw our attention to just some data facts. Māori people, we're 15% of the population in New Zealand, yet 65% of the female jail population is Māori. 65%. Māori men make up 55% of the New Zealand jail population. And so when we think about how these things occur, we have to remember, we have to remember what has occurred in the cultural context. And when we think about resources, when we think about items that we have in our possession and institutions, many of those items have been captured. through a process of colonization. And when we think about these people that are living in circumstances that are quite awful, awful circumstances that our indigenous peoples are living in, we have to take on board that people are often alienated from identity, alienated from identity. And often the only way to connect with one's history is to go to a library, to go to a museum. to go to a gallery to buy a photo. I went and brought a photo for $800 of my grandmother and her two sisters that was taken at Waitangi in 1981 because they were indigenous women sitting in an area around the marae that looked fascinating to a photographer. Do you know what I mean? And then as a mokopuna or a grandchild that discovers that photograph, and you go, gee, that's beautiful, for a moment you do, and oh, I'm gonna buy that photo. And you purchase that photo, and that's a lot of money, but how many other indigenous people have $800 to go and buy a photo of their grannies? Not many. And so I want to just take a moment to reflect on the scavenging that occurred, and how things got into institutions. Because those things are being digitised now, and before ChatGPT and Whisper and all this sort of stuff, we were already putting those things online. We were already putting those things online in this rush to digitise and the digital transition and we're all part of it. Giving it away. Giving away stuff that doesn't belong to us. Not thinking about the values and principles of those people that those things truly belong to. Now, that probably sounds like a lot in response to your question, but I wanted to draw our attention to, you know, the Flores paper. Is anyone here familiar with the Flores paper? You are, yeah, yeah. And just chime in, chime in here, because, I mean, this paper is remarkable in that it does not tell us where the data comes from. And usually when you have papers like this, it speaks to the origin of the data. So to me it's unusual in that respect. It also draws our attention to what type of decisions are made when benchmarking for marginal languages is occurring outside of the language speaker community. And what we're learning is that 50% is good enough. Not for us. We've worked hard at Te Reo Iri Rangi o te Hiku o te Ika to ensure that our accuracy is 92%, which outperforms any big tech attempt with an indigenous language. The reason for that is because we know that our culture and language has already been harmed. And one of our principles is do no harm. do no harm and we're trying to ensure that the examples that we do produce are quality examples to enable the acquisition and exposure of our people and the lovers of Te Reo Māori because it's going to take more than just us to save our languages. We need to find allies and we need to develop relationships to make strategic decisions that focus on quality, not 50% is good enough. And I say that because when you do translations in some of these big tech platforms, I wrote in, I wrote in, kia kaha wāhine mā, which in Māori that means, woman be strong. And then I was sitting with my aunties, and the translation for Kia kaha wahine mā was, let white women be strong. And for my aunties, that was problematic. And then we wrote, kia ora wahine mā. And then that one said, save white woman. And so I thought it was interesting because what that tells us is the recognition and the translation of those features of our language. Mā is white, but mā also means Many. E noho tamariki mā. Children, sit down. It does not mean white in that context. Our words have many meanings. One word can have multiple meanings depending on the context that it's used. And I just wanted to raise that to our attention because there was an incidence where Lionbridge was reaching out to indigenous communities all over the world. Iwi Radio, we were contacted by Lionbridge. This was some years ago. in 2018, offering to pay $45 American to any of our native speakers that would speak our language to a computer. This compelled us to get on with it and do it ourselves. We later got another notification telling us that there was some connection between Google and Lionbridge. But what I'm saying is we need to be aware of what's occurring and why. And if big tech is going to save us, I have a lot of questions about that. I have a lot of questions about that, because when we think about the health and well-being of our people, and we look at the diversity of those people that are employed in tech in general, there's not many Maori, Pacific, or indigenous people. So the remembering, I believe, would be very limited. Kathy, thank you. That was intense. That's wonderful. What advice do you have for collecting institutions and archives about how they play a role in our society with the massive, speech-rich collections they hold? So I think cultural institutions find themselves caught in two very difficult tensions to negotiate. On one side, you have what I would call mission-aligned data. So you have incredibly rich speech, audiovisual, cultural collections. I think I've heard you say, Keir, that you have years' worth of Australian speech. If I were a big tech company, my eyes would light up in anticipation. The other tension that you're navigating, or you are custodians of that data, the other tension that you're navigating is the funding situation for cultural institutions. And what are your options for securing different streams of revenue? Now if I were a big tech company, and you had all sorts of speech data that would make models work better, and you were in a tough financial position, that would make it an incredibly attractive opportunity to offer you a bucket load of money. for your culturally rich speech data. So I think that's going to be a tension that cultural institutions are going to have to navigate. And I think what I would encourage is some very difficult conversations now. around what position cultural institutions would take when big tech comes knocking for your data. Because I think it's a case of when, not if. And I want to paint a broader picture here of something that I'm labeling the token wars. And I'll explain why I'm using the metaphor of war. So if we think about generative AI, at a machine learning level, generative AI is based on an architecture, a structure, that uses something called transformers. And transformers work with data that has been tokenized, chopped up into chunks in very particular ways. And essentially, the more tokens that you have, the better your model is for various values of best or better. Now, what we've seen in large AI companies is basically scraping the entire public internet to tokeniser, to create tokens. And in response, we've seen sites like Reddit block scrapers. We've seen journalism websites enter into content contracts, basically so that their content can be tokenised. So what we're seeing is basically this site of conflict playing out. Actors who have incredibly rich collections of data that can be tokenized are now having to protect that data from unscrupulous actors who want to harness and harvest that data without remuneration. You've got large actors who are seeing potential returns in the AI space, trying to harvest as much data as possible. So we're seeing that conflict play out. And I think cultural institutions are coming to realise that they're also actors in the token wars. And my advice would be to have some very robust conversations about what policy positions the cultural institution will adopt in terms of Who does that data get shared with? For what purpose? And coming back to the point about data sovereignty, who benefits from tokenization? And the rematriation or repatriation of data by indigenous people is often a strategic relationship that can be developed with institutions. And I always mention this because these items that institutions are the custodians of are often representations of tribal history And that tribal history is something that a lot of people don't know about. And when we think about language technology and we think about the connection between language and culture, whilst those are physical items, they are items that often have their own story. And the stories associated with items I think are an important opportunity for collaboration, because our items often have names. They're personified. They're not just an item. They're often revered items that have been sung about and danced about, that have travelled through generations. I mention that because when we think about benchmarking, you mentioned the WISPA model. We had issues with the WISPA model because it was unclear where the data comes from. When we looked at the Te Reo Māori evaluation of FLIRS, there were only four speakers. You know, four speakers, and two were of good pronunciation, two were of poor. They claimed that they were native speakers, but they were not. And so I think as institutions, we are often the holders of that knowledge, or the keepers of that knowledge for the time that those things are with us. I mention this because if it's all being vacuumed up, We do, I believe, have a responsibility to at least record what we know. And what we don't know, collaborate with the people that belong to those items so that we can ensure that there is some record. The provocation, Kathy and Peter, to do the work internally, cultural work, the thoughtful work, the policy work, the principles work, the governance work, in advance of the moment when you are cash-strapped and someone is offering you money for the culture of which you are custodian but not owner. It is not our culture to give away. So the National Film and Sound Archive of Australia, we did some projects in this space last year and then kind of paused them to do that policy work and came up with a set of principles that are kind of under three banners of maintain trust, build effectively and publicly. It's part of this conference is actually us doing our work publicly and generate public value. And the ask for our content to turn into Whisper 5 or something clashes with basically all three of those. And so it's then a thing we can then say, well, we can't do that, because we've gone and done the thoughtful work in advance. And I really encourage other institutions to do equivalent work. I have seen other people who have come to me and said, oh, we've used your principles to make decisions that we actually didn't have a guide rail for. We need to generate our own guide rails that are grounded in our institutions in this moment. Before it's happened to us, we want to be active agents. I wanted to end on a hopeful note and ask you both to, this is Fantastic Futures, it is a futuring conference, we are talking about the present and what's coming, maybe with you Kathy and then Peter, what are your hopeful five years out, three years out in this space? What are the things that can happen or that are on the cusp of happening that we could encourage? So what I would point to here is something that is already starting to happen. I think I'm going to perhaps touch on a lot of the themes that we've talked about over the last 45 minutes and hour. One of the things that I'd like to see happen in the next five years, which the NFSA is already starting to pioneer, is really to have some national, Australian, culturally aligned, national capability aligned foundation models. So a foundation model is something like Whisper, it's something like ChatGPT, that is built with a broad range of Australian stakeholders, Indigenous, stakeholders, cultural institutions, government, industry together, because I think what we're starting to see happen, and Peter Lucas, I know that you and I have talked a lot about this, is another force of colonisation that's starting to happen. So the conversations that I hear in the AI space at the moment are very much around Australian businesses, Australian organisations need to use more AI. We need to adopt more AI. The question I hear a lot less of is where is that AI coming from? What is it grounded in? What are the values that created it? And when we start to think very deeply and very critically about that, most AI at the moment is coming out of the United States from a very particular cultural context. Whisper is American. ChatGPT is American. Quad is American. We're starting to see another process of colonisation happening, but it's coming from a very different place. So, you know, Whisper has displaced a lot of other pieces. Whisper works very well for American accents, most of them, unless you're from Boston. But what we don't have is an Australian GPT. What we don't have is an Australian whisper or an Aotearoa whisper. And I'd like to see governments, cultural institutions, start to build some national capability in this space. I think the NFSA is at the forefront of that. One of the things that, touching, going back to benchmarking, which Peter Lucas, I think you make a very good point, I had to build the data sets to test Whisper for accented speech because we don't have a lot of those data sets. Apart from mine, there's only two others in the world and they don't cover very many accents. What does it mean to evaluate an Australian speech model? What does it mean to evaluate an Aotearoa speech model? And I think we need to do a lot more evaluation work to see whether these AI tools work well culturally, whether they work well within the range of Australian context. and whether they work well for the range of Australian speakers we have. There are over 250 languages spoken today in Australia and there are very many accents. I want a whisper that works for all Australian speakers. I would like to acknowledge that Te Rewirirangi o Te Hiku o Te Ika Te Hiku Media, of which I am the CEO, is working closely with Nga Taonga Sound and Vision and Radio New Zealand to look at how we can, together, create tools that recognize a range of Māori accents, a range of New Zealand accents, because indigenous people, particularly Māori people, in my experience, speak a different form of English. And that, too, is its own accent in English. But I say this because data licensing and the discussion of data licensing for indigenous language models has been a big part of our journey. And that's because our key drivers are things that we wanted to amplify. Those key drivers include building an indigenous language economy. Those key drivers are about advancing indigenous data science. those key drivers are about strengthening the values and principles that sit behind topics like indigenous data sovereignty. And that's because When we think about those values and principles, they are values and principles that may well be relevant to the institutions that are the caretakers of the items we are disconnected from. And those principles and values are an opportunity to create a strategic decision-making framework that takes into account not just the Western perspective that we have become accustomed to, but other perspectives too. And I want to say that because for us in our technology development, we've taken into account the context of our people. We prohibit certain uses and those licensees that agree to our license for our technology, They agree not to use our speech technology for the surveillance of our people, putting more of our mothers, aunties, cousins and sisters in jail. Strangely enough, there are very few of us that don't have a first-degree relative that hasn't either been to jail, is going to jail, is in remand, or leaving jail. And so, I mean, that makes you think, what other uses do we prohibit? We prohibit the tracking. the discrimination, the persecution, and the unfairness, and anything that is inconsistent with our values and principles that we apply when making decisions. And we all hear about words like bias, but unless you are the recipient of the systematic racism that occurs in decision-making, it's really hard to understand the feeling, and the feeling is not good. So I would say that there is an opportunity. There is an opportunity for us to work together, particularly in the GLAM sector, because the GLAM sector is an entry point for learning about our identity. and identity and language and culture are key markers when we think about increasing health and well-being alongside education. Kia ora tatou. Thank you both. I remember you saying data is land, and it really stuck in my head. So everyone, data is land. Can you join me in thanking Peter Lucas Jones? Kia ora. Kia ora.
The National Film and Sound Archive of Australia acknowledges Australia’s Aboriginal and Torres Strait Islander peoples as the Traditional Custodians of the land on which we work and live and gives respect to their Elders both past and present.