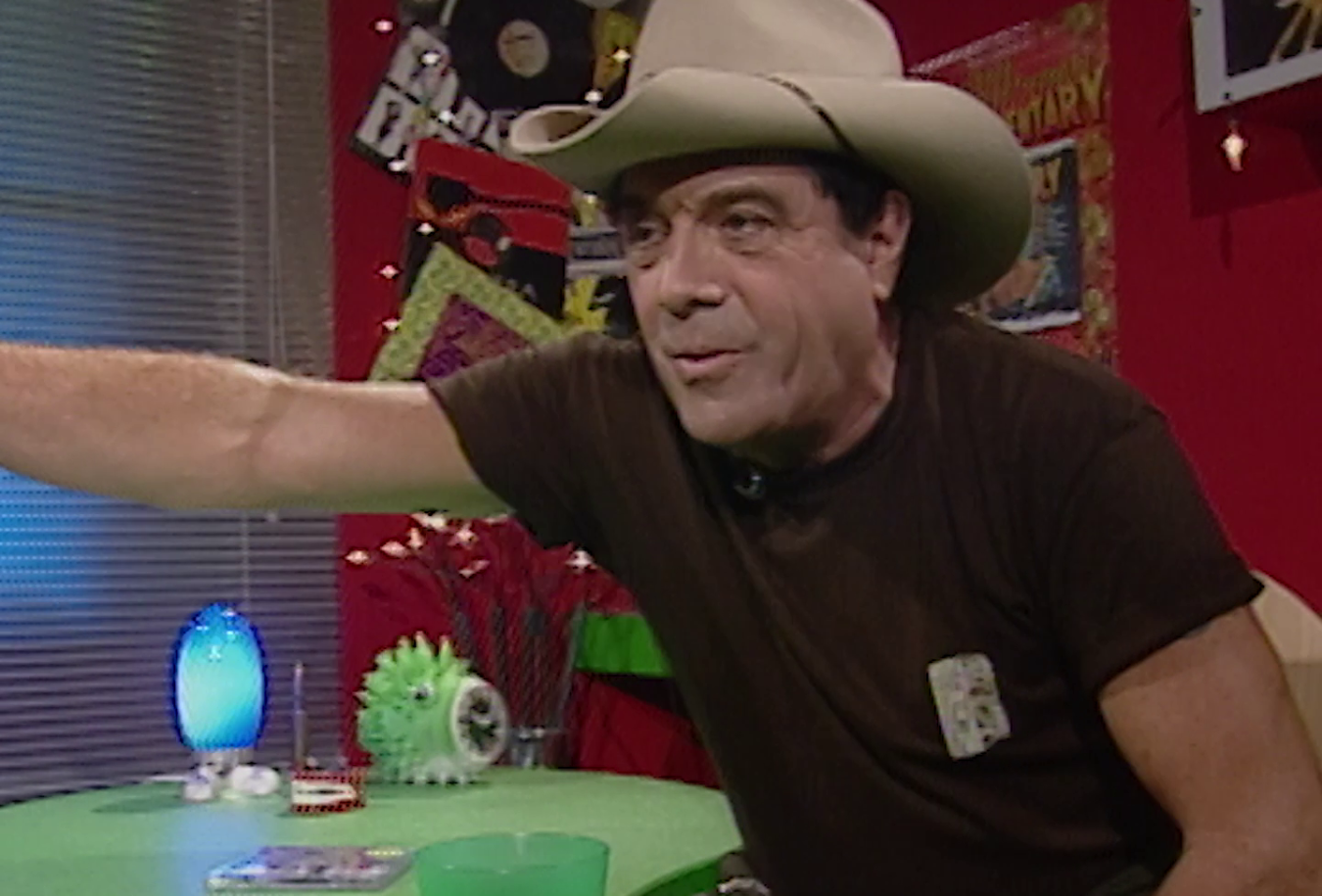
Presenter: Karen Thompson
Herbaria provide data that document the diversity, distribution and evolutionary history of life on earth, but these collections face the same challenges many museums currently contend with – a need for digitisation and findability. Karen Thompson introduces Hespi: a tool developed by the University of Melbourne that effectively identifies the location and orientation of text within an image then applies text recognition to provide a transcription.
Technology, language, history and creativity converged in Canberra for four days as cultural leaders gather for the world's first in-depth exploration of the opportunities and challenges of AI for the cultural sector.
Want to learn more about this event?
Visit the Fantastic Futures 2024 Hub
This transcript was generated by NFSA Bowerbird and may contain errors.
I don't know about you, but my brain's about to explode, so just stay with me. You've only got one or two more to go through. I'd like to share with you the work of a small interdisciplinary team from the University of Melbourne of three research data specialists in academic roles and the curator of the Herbarium. Together we created a project that began in early 2022, so before the Kraken, that aimed at improving the task of transcribing text from digital objects. In particular, we're talking about digital images of herbarium specimen sheets. So the imperative to digitize is universal, obviously, and the findability of those digital assets is heavily reliant on appropriate metadata. And I'm not talking here about enriched metadata. I'm only talking about the objective transcription of textual elements from a sheet to a digitally readable format. And this work can be a bottleneck. It requires humans to be involved. But more so, natural history collections, including Herbaria, face different challenges that other text-based collections do. Most evidently, there's more on the sheet than just text. The text can be located anywhere, usually guided by the plant morphology or by the labels that are already on the page. The text information differs. The labels evolve over time. What they're collecting evolves over time. And often one herbarium can actually be the recipient of collections over dozens if not hundreds of years that have their own way of creating data. The kind of text differs. Any way that's been used to put text onto paper we will find on a specimen sheet and often in combination. Old typewriters, handwritten in pencil, laser printers. The text is mixed language with the taxon often being in Latin, the location being in English, but not always because our collection extends to international material. So because of these challenges, OCR is not going to be successful if it's applied just to the sheet. We need another approach to create and support automated transcription. So our team created HESPI, which stands for Herbarium Specimen Pipeline, a pipeline which is just a set of steps that programmatically hang together. But don't let our current application limit you. This framework can be used for other collections. In this phase of work, we focused on the institutional labels. That's what I'll be looking at mostly here. That's where the primary data is for transcription. But we will be extending this to other elements of text on the sheets. So the two images on this slide are the result of putting these, the two images from the previous two slides, through the first step of HESPI. And that is where computer vision is used to outline various components on the sheet. the non-plant components. So a word of warning before the next slide, it does contain moving animation, so if you're sensitive to that, take care. I will be verbally describing most of what's on there. HESPI takes the input as a digital image from a specimen sheet. That is then sent through an object detection model that we've built. And Emmanuel this morning outlined one of these object detection models, which I'm very grateful for. We trained and tested that based on over 3,000 annotated sheets. The output from that we saw in the previous slide and as we're currently focused on the institutional labels, the label is snipped out and then sent through a second object detection model that looks for areas of text and it separates the pro forma from the entry text. The institutional label is also sent through a classifier which identifies whether that response text is handwritten or typed or printed. The resulting text fields then go through OCR and HTR. That resulting text then is actually checked against some of our controlled vocabularies. And that's really important for information where you have absolutely no discretion about how your species is spelt. And that then is extra gone through, extra gone through, that's right, goes through a multimodal large language model. We started this project in early 2022, the large language model we've been able to incorporate this year. And that checks everything and refines our text. So the output is the text on the institutional label from the specimen that's now in a format that can be put into a metadata in a collection system. There's an awful lot of labour involved in that. And each of these elements in the green boxes can be pulled out and replaced by an improved model. They can be retrained, they can be refined, and they can be run independently without creating any issues for the whole pipeline. So if our herbarium was to inherit another small little collection, we'd be able to take our models and retrain with a modest number of annotations to get a new model to put into that pipeline. Now, similarly. Any collection who wants to use this model can do the same. A modest number of annotated specimens use our model and create your own model to put into that pipeline. A lot of particularly small collections don't have the resources for the enormous labor to create the annotation and also the compute that's required to train these models. So to support that, we've made our model freely available. We've made our annotations freely available and we would love to see someone pick up our work, any herbaria, any natural history collection, any collection with very similar text transcription challenges. Pick up our model, train it with our model weights and I'd love to see your data and see what it comes up with. Thank you.
The National Film and Sound Archive of Australia acknowledges Australia’s Aboriginal and Torres Strait Islander peoples as the Traditional Custodians of the land on which we work and live and gives respect to their Elders both past and present.