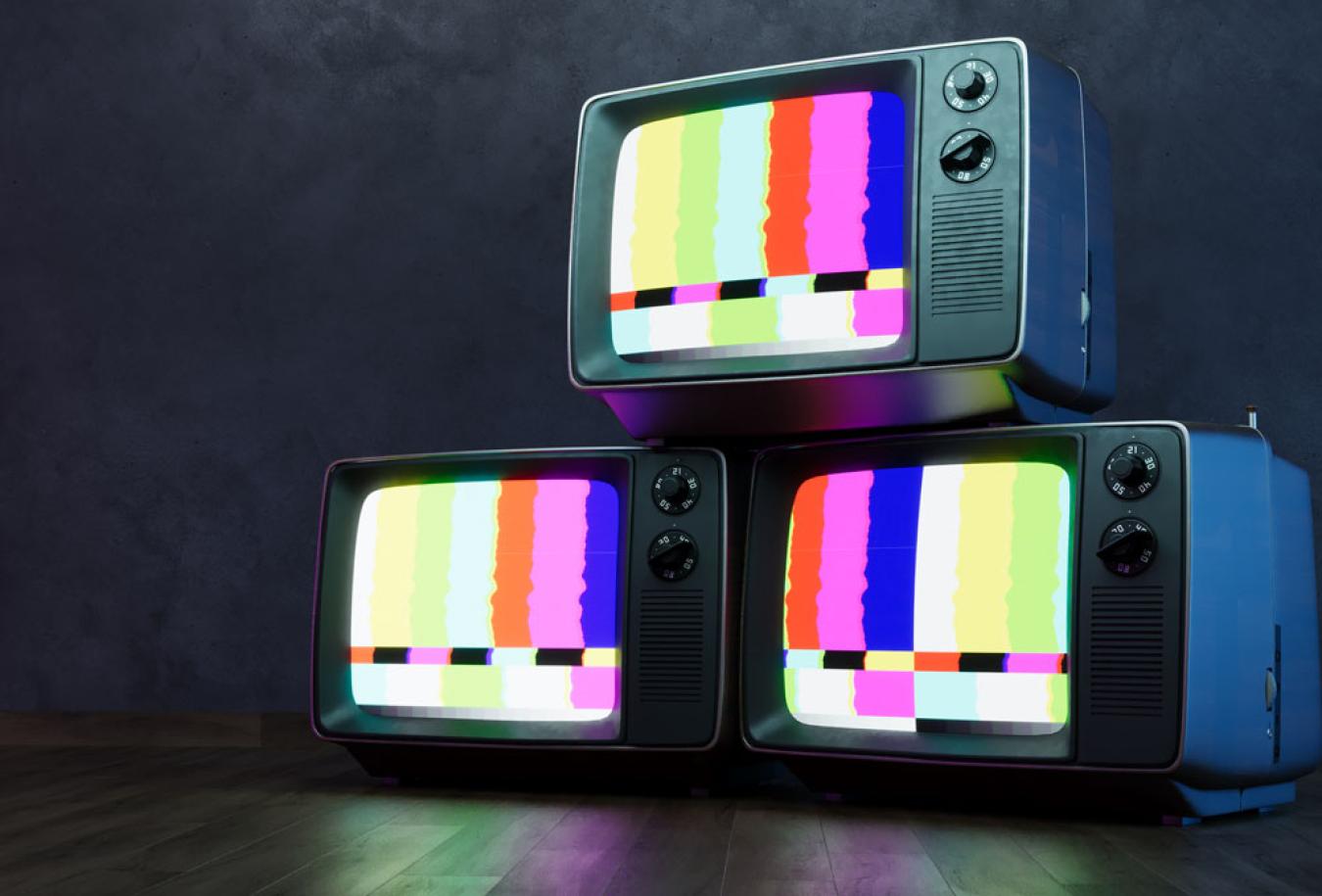
Presenter: Barbara Shubinski
The Rockefeller Archive Center initiated a research project aimed at the digitisation and subsequent analysis of analogue records from the Rockefeller Foundation collection. This resulted in the construction of a Neo4j knowledge graph database, encompassing over 80,000 nodes representing various entities and creating a visualisation of over one million relationships. Barbara Shubinksi demonstrates the potential in research and data analysis by showcasing an aspect the project uncovered: gendered assumptions within a corpus that ostensibly ignored gender, biases and institutional hierarchies.
Technology, language, history and creativity converged in Canberra for four days as cultural leaders gather for the world's first in-depth exploration of the opportunities and challenges of AI for the cultural sector.
Want to learn more about this event?
Visit the Fantastic Futures 2024 Hub
This transcript was generated by NFSA Bowerbird and may contain errors.
Thank you so much. I'm grateful to be here on Indigenous land, and I thank Auntie Violet and her people for their welcome to Country. I'm Barbara Schubinski. I'm Director of Research and Engagement at the Rockefeller Archive Center. We collect the records of American philanthropy, mostly large U.S. foundations that work globally, including the Rockefeller Foundation, Ford Foundation, and more than 40 others. American foundations represent private wealth that is held exempt from government taxation in order to fund projects in service of the public good. But it's crucial to note that what that means is decided by privately appointed non-elected persons. Our mission is to make these records publicly available, and we serve 3,000 to 4,000 researchers a year. Most of our depositors are organizations still working today, and they now produce born-digital records. But our archives hold 65,000 cubic feet and 100 years' worth of paper records. These have been used mostly by historians working narratively and harder for scholars working computationally. When asked what kind of data we have, I often say we don't so much, have data as potential data, miles of it. In January 2022, I began a demonstration project to ask what kind of usable structured data might be mined from unstructured typewritten documents. I selected 10 years of Rockefeller Foundation science grant making. In the 1930s, the Rockefeller Foundation was the world's leading funder of academic scientific research by far. The foundation launched in 1913 with $2 million. That would be about 6.5 billion in today's terms. Decisions were managed by foundation program officers who wielded enormous power by deciding where the money should go, to whom, and for what. We ingested about 12,000 pages from two record groups, Board of Trustee Minute Books and Officer Diaries, which are detailed logs of all daily activities, meetings, phone calls, and site visits. In short, between the two, the Minute Books and the Officer Diaries, we were able to bring together every dollar spent and every conversation had. In this period, it is no exaggeration to say that there are massive implications for the shape of our world. Rockefeller funded the emergence of the new fields of molecular biology and nuclear physics, including quite directly the development of the atomic bomb. With this project, we wanted to unseat the authorial hand of the program officer by looking not just at the summary story, the easily searchable famous scientists, but at the messy hole, the roads not taken, people not funded, and the full extent of intellectual networks. The ontology that we created that you see here represents the near universal dynamics of traditional grant making. Endowments earn interest. Trustees make decisions to spend that money on projects recommended by program officers. These funds go to organizations and individuals for specific projects and research topics. We ran our 12,000 pages through Amazon Textract and eventually entered our data into a Neo4j knowledge graph. The graph now consists of about 400 nodes with more than 3.7 million relationships among them. For quality control, each node and relationship is linked to the ability to view the original text from which the data came. I should have said 4,000 nodes, sorry. When we began, we used NLP tools and techniques to identify nodes and relationships. By the end of our first year, we had proof of concept, but too much noise, mostly around named entity recognition and distinguishing between people talking with each other versus people talking about other people, crucial for network analysis. That was in December 2022. Our second phase adopted GPT-4, and it was able to clean up and disambiguate so effectively that not only did it mostly solve our issues, but I decided to throw sentiment analysis into our prompts for fun. For many decades before the internet, program officers were shockingly frank in committing to paper all manner of editorial commentary about the quality of their contacts and grantees. To my own shame, I hadn't included gender in my original research plan, knowing that this era and this field was dominated by American and European men, and that women would be few. So imagine my surprise when we ran a query seeking the people most talked about in the graph, and female scientist Dorothy Rinch ranked second overall. When we pulled up all the comments gathered on Dr. Rinch, it became evident that they had a distinctly gendered quality to them. She was described as preachy, making too much of the data, receiving too much publicity, and even as a nuisance. We decided to look for the other women in the graph, but how would this be possible when the officer diaries by convention recorded scientists by their first initials plus last names or by last name only? This part turned out to be surprisingly easy. It turns out that while there was almost no regularity to whether male scientists were called doctor, professor, or merely by their last names, Program officers, without fail, noted when they were talking to a woman by putting either Miss or Mrs. in front of her name, even when said woman was in fact a doctor and a professor. Of the more than 15,000 persons in the graph, 653 are female. Percentage-wise, they receive positive and negative comments in about equal distribution to the people in the graph who are non-females. What became evident was that it wouldn't be a case of women being assessed negatively more often than non-females, but rather it would center on the actual content and context of what was said about them. we found that women were almost always assessed to whether they were attractive or not. If attractive, program officers openly speculated about their presumed higher likelihood of getting married, making them therefore a bad investment for the foundation. Even further, the program officers frequently wrote about not wanting to invest in women, even those few whom they actually called brilliant, because as women they would have no chance of going on to direct an institute or other career milestones, as if the lead science funder in the world were merely an observer of the system and not a primary agent of it. While these comments have come up anecdotally in prior research focused on particular biographical narratives, until automation and machine learning, it was hard to locate and assess them in the aggregate, not to mention to connect them to other key aggregate numbers. Of the 653 women in the graph, two received grants as principal investigators. There are 334 major grants made during this time period and multiple hundreds of smaller grants in aid. Nine individual women total received funding in any form. This project wears two hats. It's both a research project genuinely concerned with the content of its findings and a demonstration of technical possibilities for my institution. As such, it's shown us the transformative potential of tools like large language models in historical research, helping us envision new avenues for understanding, and even more so, for giving researchers what they might need to interrogate archival materials toward a more nuanced and inclusive historical narrative. While some of this may seem par for the course for the era that I'm talking about, I would suggest to you that the kind of commentary we see in the officer's diaries and the kinds of hard-to-dig-out disambiguations about gender, race, and other characteristics that are not intentionally part of the record, as well as the kind of candidness of these records might have very different implications in other time periods. For example, the U.S. Civil Rights Movement or the 1960s decolonization efforts in African nations of which foundations played very, very primary roles. So I think the project has a reach further than simply its demonstration, but this is our first start. Thank you so much.
The National Film and Sound Archive of Australia acknowledges Australia’s Aboriginal and Torres Strait Islander peoples as the Traditional Custodians of the land on which we work and live and gives respect to their Elders both past and present.