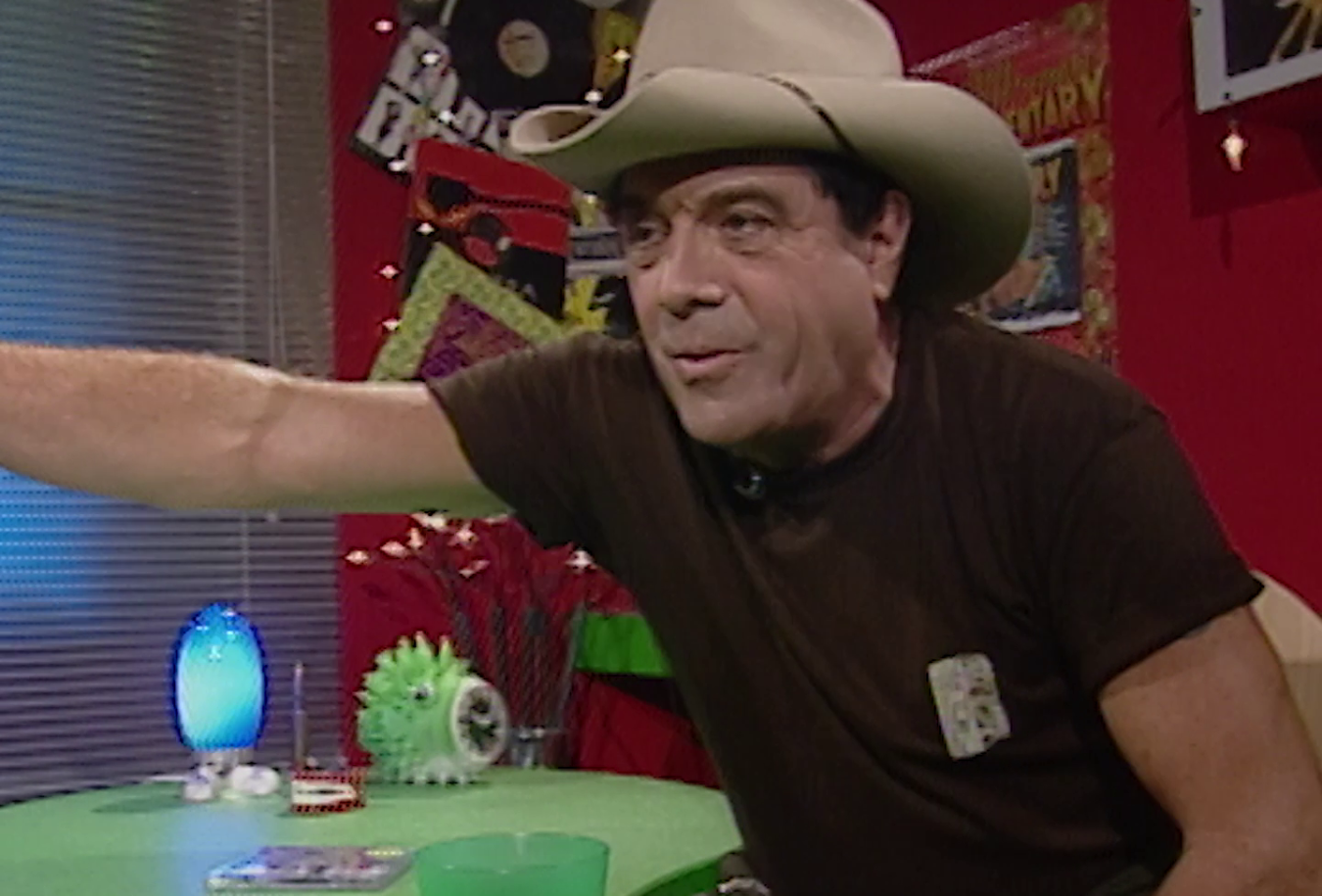
Presenter: Neil Fitzgerald
For around the last 30 years the British Library has digitised its collections. Historically, this work was undertaken not from core grant-in-aid funding but via external sources including research, philanthropic and commercial. Neil Fitzgerald explores potential approaches available to overcome barriers to applying state-of-the-art research, including collaboration via open frameworks and mutuality – an alternative path to reliance on purely commercial options that enables everyone to contribute to and benefit from the value chain.
Technology, language, history and creativity converged in Canberra for four days as cultural leaders gather for the world's first in-depth exploration of the opportunities and challenges of AI for the cultural sector.
Want to learn more about this event?
Visit the Fantastic Futures 2024 Hub
This transcript was generated by NFSA Bowerbird and may contain errors.
Thanks Basil, it's very nice to be here. So I'm going to talk about adoption within the British Library. There's been a lot of discussion and excitement, fear, concerns, delete as appropriate, about the implications of AI machine learning on the wider GLAM sector and our users, especially following the Gen AI hikes. And I was struck by the parallels with past discussions with mass digitization, and I wondered if there were lessons that we could learn from then to now. And I'd be interested to hear your thoughts afterwards. The presentation I'll give today will include a lot of input from people across the British Library, particularly the digital research team, and you'll see them on screen a bit later. So just some background, the BL is one of six legal deposit libraries in the UK and Ireland, along with National Library of Wales, National Library of Scotland, Oxford, Cambridge, and Trinity College in Dublin. We've been involved in a range of digitization activities using a number of approaches for many years, and we're now also active in the AI machine learning space. The BL is also an independent research organization, which means that we can bid for research funding in our own right. And due to the breadth and depth of our collections, we're considered attractive to work with across the research and commercial sectors, previously for digitization and now in the AI space. So for around the last 30 years, the British Library has digitized its collections. Historically, this work was undertaken not from core grant-in-aid funding, but for external project-based funding. And that included research, philanthropic, and commercial. It's only in the last few years that we've had a permanent digitization workflow team in addition to our longstanding imaging services department. The initial focus of this workflow team has been dealing with legacy digitization and the focus of that team now is on improving end-to-end workflows and the development of automated processes. And in regards to the volume of digitised content, we've probably done about 3% of the collection, and that's across a range of content types. And that includes about 865,000 books, 83 million newspaper pages, 6 million sound recordings. And our biggest digital collections are actually born digital content. So this includes material we receive under non-print legal deposit. And from that, the UK Web Archive is now our biggest digital collection with billions of items. We also record all free-to-air TV news channels in the UK, 24 hours a day, 365 days a year. And we also have done a project called National Radio Archive where we have a selection of local, regional, and community radio stations which have been recorded and run through speech to text. So starting with boutique digitization of our treasures, unique manuscripts, often visually impressive with the end goal presentation online. Drivers were cultural restitution and wider public interest, cultural reunification, for example, our International Dunham Project and Codex Sinaiticus. Focus was on small-scale and high-quality showcases, often with recataloging metadata improvements, one of the main outcomes. Often it was said there was only one chance to capture these items, although compelling new technology advances was an exception to this rule. And then encouraged by the prevailing government ethos of the day, the libraries 2001, new strategic directions plan included an enabling strategy of using partnerships to achieve more by working together with others than we could do on our own. So this drove digitization of materials for other purposes, including partnerships for commercial partnerships that were for the family history market. And also, the Microsoft Digitalization Project, which was around 65,000 books, all 25 million pages in 19th century content. And content from that particular project really drove much of our early BL labs work. And the lab's approach really unlocked a huge amount of creative reuse of our collections. And the Glam Lab's network has grown internationally and has been a really good force for change within lots of cultural heritage organizations. In parallel, there were a number of public partnerships, and these were to help drive research agendas and support higher education. The key thing with both was the importance of finding partners who wanted to establish a long-term partnership working approach. I must stress, though, that until there was a critical mass of digitization activity across both the public and private sectors, there was little incentive for researchers and suppliers to develop tooling and solutions that could sufficiently work well with historical collections. These general principles developed in the early 2000s to guide our large-scale mass digitization projects are still relevant today. They also helped inform our contributions to later internal initiatives such as our data strategy and other even later external contributions to initiatives like collections as data. And with a bit of tweaking could also apply to other contexts like AI. So following this extended period of often fragmented digitization activity in the UK, and after long periods of lobbying for a more coordinated approach, UKRI and the Arts and Humanities Research Council, and these are the key UK research funders in this space, launched in 2020 this major five-year, 18.9 million investment program with the intention to take the first steps towards creating a unified virtual national collection by seizing the opportunity presented by new digital technologies. The intention of the foundations within the program was to generate evidence and policy recommendations to inform future development of the UK infrastructure for digital collections. The program, like everything else, was significantly affected by COVID, and it still remains to be seen what the long-term impact will be. Institutional adoption of new technologies and methods can be disjointed. There are many reasons for this, including required organizational practice change, which in the UK has been compounded by the tightening fiscal environment over many years. Even when evidence action has been justified and accepted, implementation requires sustained effort. For fields such as AI and machine learning that gain through such rapid change, this can create high perceived organizational risks that need to be managed in an appropriate way. We can draw on other relevant organizational experiences where we've built up the knowledge over time to inform our approach. It's also really important to highlight within our organizations that we've been successfully applying computational machine learning techniques for many years, harnessing new technologies for the benefit of users and the communities that we operate in. So with the explosion of digitization initiatives in the early 2000s, we set up an internal coordination group called Digital Initiatives and Monitoring Group, called DIAM, to support the development of knowledge and new workflows across the organization, sharing lessons learned, and to interface with external networks active in this space. Work across the group helped to develop our approach working with commercial parties. Our key licensing in and out principles and over time helped make the case for the importance of not hollowing out organizational capability and cohesion through outsourcing to third parties. I'd argue that these aspects are now even more important in the current AI cycle. For individuals, the organization and the sector as a whole, it's really important that we enable an experimental mindset whilst providing appropriate guidance and support. We've already collectively invested a lot of time and effort in developing workflows that reflect our values and highlight the benefits of working in the open. For example, IIIF that we've heard about in other presentations. This came out directly of the explosion in digitized content, and the collection holding institutions decide to find a better way to support the needs of users and organizations across a range of domains. The work to develop use cases and cookbook recipes for its application in the AI and machine learning space is underway, and you're invited to join those working on developing them. So to help drive research and development in the digitization space, initially for improving OCR, during 2008 and 2011, we built this EU-funded research impact dataset containing around 500,000 images from collection-holding institutions across Europe. This matched their digitization priorities at the time and looking into the future. Around 10% of these images had corresponding ground truth, so 50,000 pages, to support innovation. Before this, data was made a fable. Researchers found it very difficult to obtain content that highlighted real-world problems. Access to data to support R&D continues to be a significant issue. Recent papers have called for the creation of commons datasets to drive wider benefits in this space, including this one, although I don't think they fully recognize the potential of the volume of how to copyright digitized contents that will be coming out of contract shortly. If we look at the early Google partners, for example, there'll be a moving wall of collections if able to support a public good approach. A number of cross-sector initiatives are starting to emerge that aim to lower the barrier to entry for everyone, increase public value, mitigate the negative environmental aspects of AI and machine learning through shared approaches, including the reuse of training data and models, and of which are owned by the communities themselves. It's in our shared interest to ensure there's a strong public goods alternative to commercial options. Collection-holding institutions have a long track record of brokering competing demands in this space, including moral, ethical, and relational, in addition to copyright. Although we've heard over the last days there's more work to do. There's been much discussion and lobbying for changes over many years, both for competitive advantage and also to try and mitigate issues with the current situation. As we've seen in the past, for example, the EU often marks an extended collective licensing failure. Making copyright more complicated will not deliver outcomes that benefit all of society. There's already an exception in UK law which allows for computational analysis, and this includes AI, for non-commercial research. Other jurisdictions are more permissive. Legal frameworks that are scalable will improve compliance. Bad actors will do whatever they want, and we should not penalize those working as good citizens. And currently in the UK, there's a consultation on extending or providing more flexibility within existing frameworks to support computational and AI research. it's unclear what the outcomes will be. So, here's the digital research team and the universal review team. This team was established in 2012 in response to a strategic review of the library's then scholarship and collections department, with one of the key aims to better support digital scholarship. The library's collections and scholarship is international. we will not be able to operate effectively, and without our global connections across academia, other GLAM organizations, and participation in consortia and communities across a range of mutually beneficial shared interests, such as this one, and most importantly, with our users. So one example of the work we've done is in the field of automatic transcriptions, initially OCR and more recently HDR. The library's interest in this area started following two large newspaper digitization projects funded by JISC undertaken in early to mid-2000s. These showed the importance of usable OCR for text and data mining research methods and led directly to the Pan-European Impact Project, whose aim was to make digitization better, faster, and cheaper, especially for the type of historical materials found in memory institutions. And as we've seen from other presentations during this conference, OCR is still not a solved issue. We've continued to engage with different OCR and HDR initiatives, especially for low-resource languages present in the library's collections. One successful strategy to accompany this has been competitions that invite researchers to tackle the challenge of generating accurate, scalable transcription of historical texts. As a collection holding and independent research organization, we're interested in both advancing the state of the art, but also translating that research into operational and production workflows. Today, the library mainly uses machine learning approaches for OCR and HTR, but in the past, we have used off-the-shelf commercial products. So, the platform we predominantly use now is TranscribeUS, and this is a comprehensive platform for managing, transcribing historical documents. The software is being developed and maintained by European Cooperative Society, which came out of two EU-funded research projects. The BL is one of the founding members and the shareholder in Transcribers. Transcribers offers state-of-the-art OCR and HDR technologies, enabling non-technical users to transcribe documents accurately. The software can automatically detect and analyze the layout of documents, distinguishing between different text regions, such as columns, paragraphs, and lines, as well as recognizing tables and images. Users can train custom models specific to their document collections, improving accuracy for particular handwriting styles or print types. It can be trained to recognize most languages, The software includes features for uploading, organizing, managing large collections of documents, making it suitable for both small projects as well as extensive archival work. It also has IIIF support, which minimizes data management issues. Transcribers also provides comprehensive user support, including webinars and other community activities. So looking at some of the specific projects, so we have quite a diverse collection, including Bengali books. And this was our original flagship transcriber's work, and we did this as part of an AHRC-funded project called Two Centuries of Indian Print. And this focused on the 22 South Asian languages that are present in our collections, starting with Bengali. Work was done by an ex-colleague, digital curator Tom Derrick, in collaboration with partners at Jadavpur University in India. After experimenting with different software solutions, Tom trained transcribers to automatically transcribe early Bengali printed books. He established a workflow monitored by Power BI dashboard so people could work on this collaboratively. Using this pipeline, we've produced automated transcriptions for 1,200 Bengali books. BL initiatives for Arabic handwritten text recognition started in early 2018 by Nora in the digital research team and continued by Adi. We have almost 15,000 Arabic manuscripts in the BL from the 8th to the 19th centuries from many different regions and countries. The HDR work targeted specifically scientific manuscripts digitized as part of the British Library Qatar Foundation Partnership, and these items are available via the Qatar Digital Library. We organized competitions in 2018-19 in collaboration with the Alan Turing Institute and the Premier Research Lab. The aim was to find an optimal solution for accurately and automatically transcribing historical Arabic scientific handwritten manuscripts. And this project really demonstrates that subject matter experts are crucial to progressing the state of the art, and they need to be actively involved in these types of projects. So looking a bit closer at the specific changes when it comes to OCR and handwritten text recognition, our competition participants used an example dataset to train their systems and then tried their software on an evaluation dataset to produce OCR results in XML format. These results were evaluated by the premier research lab and winners were announced. We host the ground truth competition datasets on the BL research repository, and these continue to be downloaded and used by researchers. And we also share out the Arabic hammer and text recognition transcribers model on request. So, moving on to Urdu. So, again, using transcribers, we looked at automating the transcription of early printed Urdu books. To teach the system how to read Urdu, we needed ground truth, a set of at least a few dozen perfectly transcribed pages. So we crowd-sourced transcriptions using transcribers, and at the end of the initiative, we had almost 70 pages of fully transcribed content by volunteers, which was a really good outcome of digital engagement. More work is needed to continue development of an Urdu training model, by the end of which we'll also be able to process our digitized Urdu books, which currently are around 400. Moving on to Chinese, we expanded our efforts to Chinese collections and automating recognition of historical Chinese manuscripts. Our focus was on manuscripts digitized as part of the international Dungbom program, a collaborative program that digitizes and makes accessible online collections from the Eastern Silk Roads. We've collaborated with Colin Brisson, and he was in the process of developing training models for segmentation and text recognition using a platform called eScriptorium, which is an open source product, and was stronger with working with Chinese than transcribers currently. We hosted a PhD placement student last year, Peter Smith, a third-year PhD student at the University of Oxford. He joined the library's digital research team for three months. His main remit was to work with Colin to improve his training models. Peter worked closely with him on four aspects of the HDR process, image selection, binarization, segmentation, and transcription. So looking at this in more detail, binarization is a common preprocessing step before doing OCR or handwritten text recognition, and it can greatly improve recognition accuracy. It's basically the process of converting a colored image into an image which consists of only black and white pixels. There's variations in level detail that are captured by the binarization process. And you can see the varying impacts of staining and discoloration on the page, as well as the density and color of the ink. And yeah, so in those red circles there, you can see the differences between the original text and the binarized image. We then move on to segmentation. And so a significant part of Peter's work was dedicated to improving Colin's segmentation model. And this automatically assigns text regions and lines. And so you can see here the results of the automated segmentation managing to identify when a single column of text turns into two columns. Automated segmentation can have issues, especially when text is written in red or when manuscripts feature interlinear annotations. Peter maintains a log of issues that he came across on binarization segmentation and shared those with Colin. And the substantial piece of work that Peter then did was actual transcription of the text. And he ran into issues where some of the characters did not have Unicode representations. So an approach had to be agreed on how to handle that. And Colin made some recommendations which were then followed. So this has improved the model, and so any e-scriptorium users out there will benefit from this work. And it just shows the power that collection holding institutions can have. They can have a real amplification effect by releasing datasets and doing this specialized work with researchers. And thinking about British Library operational requirements, ConverterCard is one of our projects that we have worked on for a period of time. And this makes really good use of automatic text recognition technologies. So the challenge here was that the library still has hundreds of thousands of catalogue cards and many of these only exist in physical form. So the intention is to convert these so we can include them in the main electronic catalogue. So we wanted a semi-automated solution for this. Previously, work in this space was done with the help of crowdsourcing, but we've now developed a workflow to extract the text using transcribers and then automating the records matching process via the OCLC RollCat APIs. Harry Lloyd, who's a research software engineer in our team, created a web app to sort through the OCR cards and find the correct record matches for them. So the web app that he created was based on a platform called Streamlit, which we heard about in a previous presentation. So it's an open source Python framework for data scientists and AI and machine learning engineers to deliver interactive data apps. The OCR text is used to search and retrieve online catalog entries, which we can then derive them into our own catalog. So, future plans include integrating automatic text recognition into business as usual at the library. So, we've already started this process. And we want to be able to integrate transcribers as well as other open source software into our post-digitization workflows. And our vision is that automatic text recognition becomes the default phase for all of our digitization projects. And to do this, we've recruited a dedicated digital curator for initially OCR and handwritten text recognition, but speech-to-text is also on our roadmap and work is already underway on that in our sound archive. So this would be a really important step for us, making these processes business as usual for improving access to library collections for different types of users. Thank you.
The National Film and Sound Archive of Australia acknowledges Australia’s Aboriginal and Torres Strait Islander peoples as the Traditional Custodians of the land on which we work and live and gives respect to their Elders both past and present.