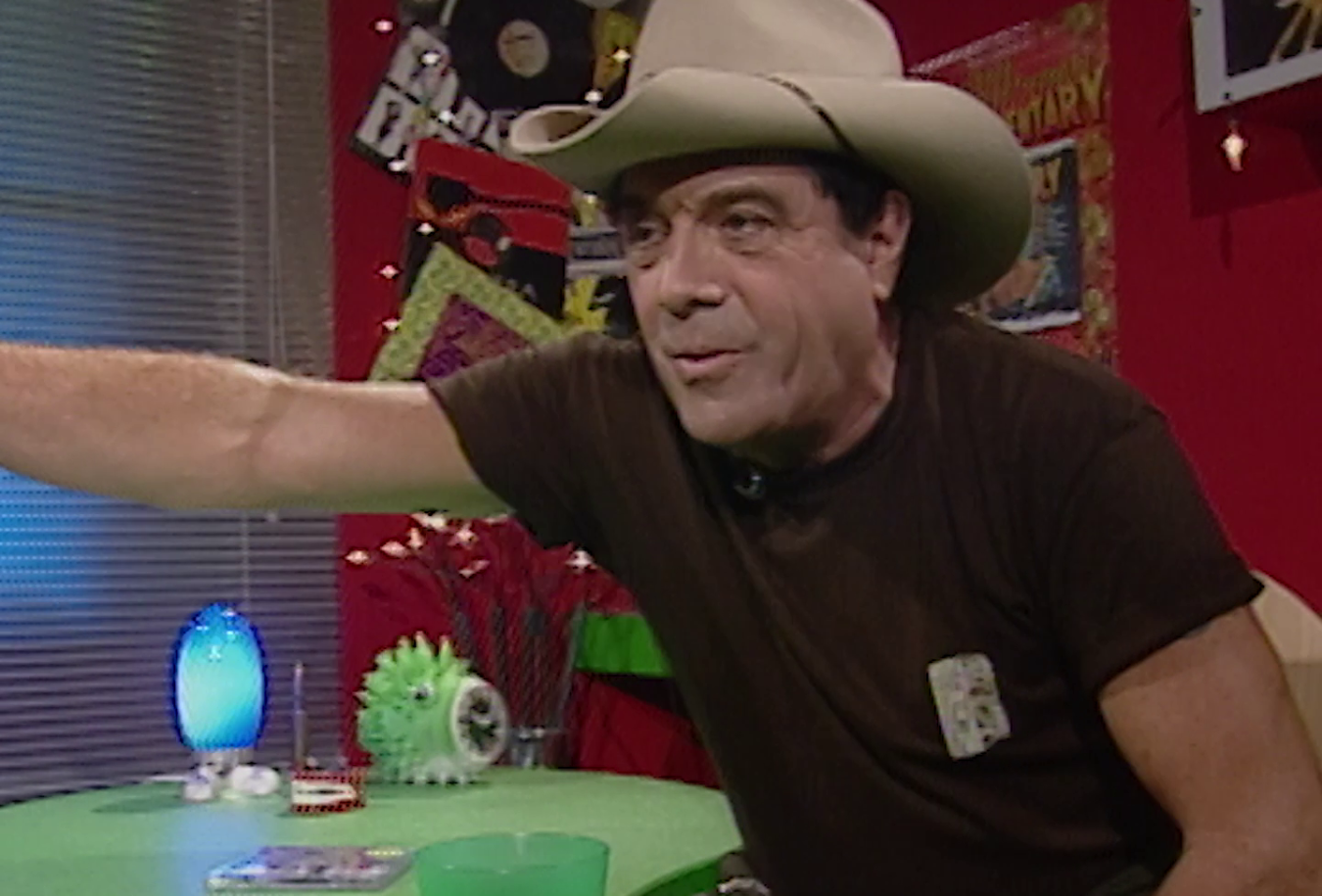
Presenter: Emily Pugh
PhotoTech is a four-year project headed by the Getty Research Institute, which focused on using AI for both art-historical research and metadata generation in relation to digital image collections. Based on the outcomes of this project, Emily Pugh provides an overview of the current approaches to image searching as they exist in the field of cultural heritage, showing the disconnects between these approaches and those required by an environment of AI-enhanced search.
Technology, language, history and creativity converged in Canberra for four days as cultural leaders gather for the world's first in-depth exploration of the opportunities and challenges of AI for the cultural sector.
Want to learn more about this event?
Visit the Fantastic Futures 2024 Hub
This transcript was generated by NFSA Bowerbird and may contain errors.
Hello, everyone. Before I start, I also want to emphasize, as Emmanuel did, that this is really collaborative work that I'm presenting, and you'll see names of my collaborators on my last slide. So today I'm going to share some insights from a user research project sponsored by the Getty Research Institute, where I serve as principal research specialist focusing on digital art history. This user research was part of a larger effort called PhotoTech, a project centered on the digitization of a large portion of the GRI's art historical photo archives. One primary goal of PhotoTek was to explore the potential of machine learning and more specifically computer vision or CV. First, as a method of generating collections metadata to power search and discovery. And second, as a tool for art historical research. The user research activities that we undertook from 2020 to 2022 were designed to support the first application, that is CV for the generation of collections metadata. Since you can use computer vision to generate all different kinds of metadata, we wanted to find out more about how and why users search for images to understand which types of metadata we should be focusing on. But user research was also to us an opportunity to assess scholars' attitudes towards computationally generated metadata. We planned a program of user research that included three components. We hired a user research consultant to hold workshops and interview scholars about their research practices. We held focused group style meetings with a cohort of scholars from the 2020-2021 GRI Scholars Program. And we designed a quantitative survey that was completed by about 500 respondents. And so today I'm going to focus on what we learned from that quantitative survey. However, the exploration of CV as a research tool ultimately yielded some compelling insights into the question of image search as well, which I'll touch on briefly at the end of my presentation. Overall, our research revealed on the one hand certain tensions between the current culture of image search, that is how people search for images now, and the kinds of approaches to search that those of us in repositories are seeking to support in the future. On the other hand, the research also revealed that the disciplines of art and architectural histories are evolving in ways that open up new possibilities. The survey we designed, which we did working closely with the Getty's head of audience research, Tim Hart, sought to capture information about the contemporary image search practices of art and architectural historians in the U.S. In addition to soliciting basic information from the respondents, such as time in the field or current position, the survey asked a number of questions about the use of photo archives specifically, including what kinds of research scholars used such archives for, the categories of information they relied on in their searches, which and what kinds of search engines or repositories they used, and how they managed their own image files. We targeted those working in the disciplines of art and architectural history, but we did We think these insights are relevant more broadly to the search practices of scholars working in other disciplines as well. So I'm going to focus on four insights that seem particularly relevant to AI-generated metadata. While we expected that responses from younger researchers might evidence the impact of digital technology more, we found that age or time in the field is not an influencing factor on search behavior or attitude towards digital approaches to research. The survey suggests that such practices are shaped how art historians are trained to conduct research, as well as the types of search tools that they routinely use. In fact, art historians' approaches to search are informed and perhaps limited by their familiarity with traditional approaches to description and cataloging. That being said, we also recognize that about half of our respondents reported 16 or more years in the field. So we need a bit more research to kind of examine these attitudes. Now really this should say granular subject description is perceived to be essential. In general, respondents to the survey reported that they valued granular information about subject matter as very desirable. For example, object types such as painting or drawing was deemed important but the more specific information about artwork material or technique, oil on wood, oil on canvas, et cetera, carried almost equal weight. However, this contradicted the records of actual searches scholars have done of the GRI's photoarchive database, which reflected broader, more high-level approaches. These findings suggest a disconnect between the traditional categories of description and search, such as single author, dimensions, national origin and subjects like Christ on the Cross, and how people actually search, which hews more closely to how a Google search operates. Our assumption is that art historians have internalized traditional categories of archival description that typically appear in collections catalogs. As a result, when asked how they might search, their answers are not informed by all the ways it's possible to search. particularly using the kinds of novel metadata CV might generate. Finally, it should be noted that traditional categories of description, many of which are based on European art, well, in terms of the Getty's collection, and especially painting, are not meeting the needs of a growing proportion of these scholars, as evidenced by comments like these. In fact, scholars who research topics outside of European painting and sculpture represent the fastest growing part of our discipline. Indeed, the survey revealed that art historians use an increasingly wide array of photo collections, including not just art historical photo archive collections, but commercial collections, photojournalistic collections, ethnographic photo collections, among others. Furthermore, our findings clearly indicate that researchers do not distinguish between different types of digital image collections. There's a real degree of ambiguity around terms like digital collections, image collections, photo archive, image database. For example, when asked what photo archives they used, at least one respondent replied with the name of a historical newspaper database. This is, of course, not incorrect, as such a database would be comprised of photos of newspapers. However, it points to the need for contextual information that conveys to users the unique conditions of the repositories or collections they're searching. The interest in granular descriptions suggested to us that there was value in pursuing techniques of computationally generated metadata, including computer vision. Our findings with regard to awareness of and attitudes towards such approaches offer further encouragement, but also some caveats. The survey outlined five scenarios, three of which entailed computation, and asked respondents to rate the trustworthiness of the information provided in each, which we noted had not necessarily been reviewed for accuracy. Respondents were asked to select either do not trust, hesitant, trust for each scenario, or could reply, I'm not familiar with this approach. The responses revealed that art historians are by no means opposed to computationally generated metadata. Specifically, very few rated any of our suggested computational approach scenarios as do not trust. Rates of hesitant to trust were higher around those forms of computation that required the machine to make an inference about an image. For example, respondents rated the scenario St. Sebastian identified in an artwork by a computer vision algorithm as 18% do not trust, 51% hesitant, and 12% trust. Whereas they rated the scenario an annotation with provenance information captured from the photograph mount by OCR as 4% do not trust, as compared with 18, 39% hesitant, and 36% trust. It's important to note, however, many reported that they were unfamiliar with the three types of metageneration processes we mentioned. 51% were unfamiliar with computer vision, 33% unfamiliar with crowdsourcing, and 35% with OCR. Now, here's where I have to point to the age of the survey. You know, we did this prior to 2022, really. So I would imagine more people are familiar with these processes now, but that doesn't necessarily, I think, translate into sort of deeper knowledge. There was a clear relationship, in any case, between lack of familiarity and perceived trustworthiness of search results, as you might expect, with participants expressing the most concern about methods that were unfamiliar and or which the method used was used to generate new data rather than utilizing existing data created by librarians and archivists. This issue of trustworthiness and how to gauge it was articulated by respondents in their attitudes towards data provenance. All respondents, regardless of their years of experience, placed a high value on data provenance. All respondents, regardless of their years of experience, I'm going to say the same thing again, we surmise that as part of the diversification in image and object collection types within art history, as well as the rise of remote access, scholars are increasingly interested in the provenance of archives and data. That is, they want to know who created these collections, for what purpose, and how they've been processed by the owning repository. With regard to computationally generated metadata specifically, the majority of respondents, over 80%, want to know if metadata was generated by computer vision or OCR in search results or an item record. This is a particular challenge since, as I noted, respondents also reported an overall lack of familiarity with such approaches. These four insights suggest to us that as part of the work of figuring out which metadata we should be generating using AI, We need to consider ways of communicating to users what they're looking at, where it came from, and generally to provide contextual information that allows them to critically evaluate the information presented to them in search interfaces. For those in repositories providing access to this metadata, it will be important also to acknowledge the influence of older, more traditional approaches to search and how these coexist with search through, for example, Google. Innovative approaches to metadata will not help users if they're not aware of new possibilities for search terms. And new approaches to metadata generation will not lead to more effective searches if they're not considered within the culture of how and why researchers look for images. While surfacing these challenges, our user research also revealed the ways we could intervene to resolve the tension between the current culture of search and future search scenarios that are driven by AI-generated metadata. So I want to end with an insight about search gleaned from the research project associated with PhotoTech. This project called Photography Unbound focuses on a corpus of about 30,000 images drawn from photographic albums in the collections of nine repositories of the US, Europe, and Asia. The project was designed to test the relevance of CV as a tool for art historical analysis rather than collections metadata generation. And some of my colleagues actually insisted that CV for research was so different as to be irrelevant to questions for CV about creating collections metadata. And I sort of understand that and think it's partially true. However, we learned some interesting things that I think are relevant to search. Working with a CV consultant, the research team was able to refine a custom-made CV algorithm to reliably differentiate between living figure and sculpture or relief within our corpus. In addition, we decided fairly early on in the process to exploit the ability of the computer to calculate coverage, or pictorial real estate given over to figures. Sorting by this facet yields some predictable results. Conventional portraiture rises to the surface, but it also reveals photographs such as artistic allegories, anonymous anthropological types, and contemporary medical subjects, images that had use values that ranged from the innocuous to the insidious. This view onto the images challenges and complicates the art historical genre of portrait. This insight prompted us to consider whether this person coverage approach could be a strategy for powering image search. It's hard to imagine how this would work. I mean, would people really want to search this way? I don't know. But it has proven useful as a thought experiment at the very least. While users express interest in granular information, and many of us are trying to figure out how to generate it, what about very broad or high-level approaches? What about focusing not just on clarifying what has been ambiguous or correcting bias, but exposing ambiguity and bias? In regard to image search, person detection seems to bear in particular on current movements towards reparative archival description and inclusive cataloging. While person detection should by no means be used as a substitute for inclusive cataloging, it could complement these efforts as a generalizing counterpoint that enhances discoverability. On a practical level, the tendency towards specificity in inclusive cataloging necessarily entails the expansion of how many categories users need to navigate. For users specifically interested in finding photographs of people, using person detection data as a first step in browsing may expedite searches by reducing the subject terms to shorter, more legible lists, potentially making more specific terms easier to find. Moreover, as institutions pursue the work of inclusive cataloging, person detection data that is comparatively easy to generate can at the same time ensure that depicted people presented in collections catalogs as, quote unquote, natives or slaves, are also presented as people. Thank you.
The National Film and Sound Archive of Australia acknowledges Australia’s Aboriginal and Torres Strait Islander peoples as the Traditional Custodians of the land on which we work and live and gives respect to their Elders both past and present.