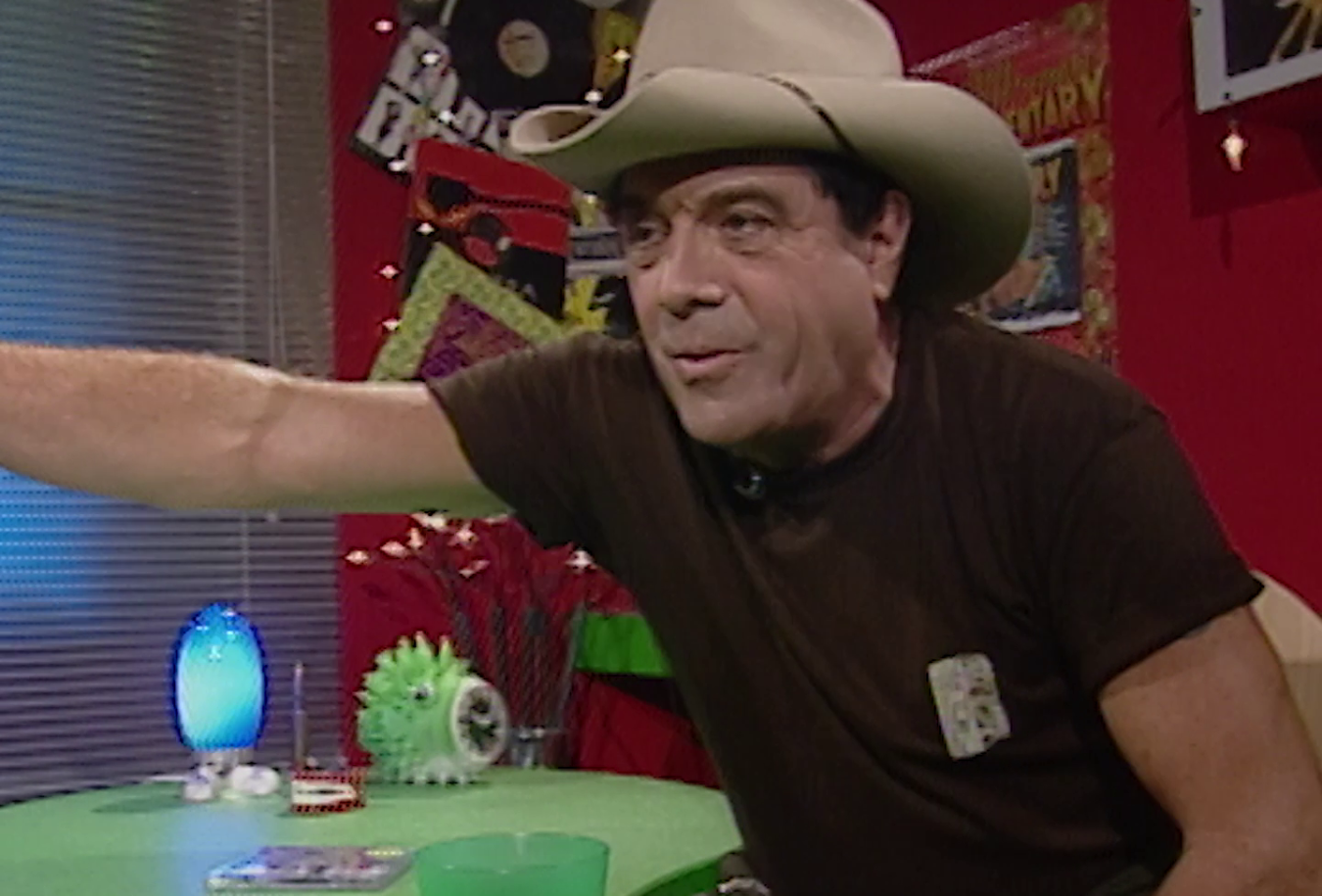
Presenter: James Smithies, Karaitiana Taiuru
Despite the growing use of large language models as expert chatbots and research assistants, there have been no obvious or accepted ways to evaluate their quality as research tools. The AI as Infrastructure (AIINFRA) project aims to develop resources to resolve that situation, including a test framework and prototype AI research tool, with a focus on transnational collaboration, Slow AI, and Indigenous design principles.
Technology, language, history and creativity converged in Canberra for four days as cultural leaders gather for the world's first in-depth exploration of the opportunities and challenges of AI for the cultural sector.
Want to learn more about this event?
Visit the Fantastic Futures 2024 Hub
This transcript was generated by NFSA Bowerbird and may contain errors.
Right, hello. This is a trailer for a project that has only just started. It's a trailer rather than a movie. And we've actually had an important advisory board and working group just on Monday, so things have evolved. But this will show you the shape of the project and direction of travel. Right so I haven't got my glasses on but I'd like to acknowledge Ngunnawal and Ngambri people that were on the land of Ngunnawal and Ngambri people for this project as well as this talk and I'll hand over to Karaitiana as well. Yeah I also just want to acknowledge country and the the indigenous peoples of this land. I also, just adding to that, my cousin's acknowledgement of country earlier today. So my acknowledgement comes from down the South Island of New Zealand. Ngā mihi. Thank you. And it's useful to point out as well that my acknowledgement of country is on behalf of the United Kingdom as well. That's a transnational project. I spent the last eight years in the UK. So we're speaking for Aotearoa, Australia, and the United Kingdom. So in terms of overview, I'll give you a quick overview and then Karaitiana will take over to talk about some of the data sovereignty and indigenous aspects of the project. First, I'll talk about what we're doing and how we're going to do it, and some emerging questions around AI, data sovereignty, and particularly evaluation of large language models and retrieval augmented generation. I'll give you a bit of a sense of some of the evaluation directions that we want to take in relation to AI and research, AI indigenous. There are lots of other sort of vectors that we want to look at as well, but those will be the two that we'll focus on today and there won't be questions. So background, project overview. The project will run from 24 to 26 with ANU Futures funding. The partners are quite broad. It will be hosted by our new ANU Haas Digital Research Hub, the Australian Parliamentary Libraries involved, National Library of Australia, Aotearoa New Zealand Department of Internal Affairs who are responsible for the New Zealand GLAM sector as a whole. UK National Archives, UK History of Parliament Project, ANU Library, King's College London, and indigenous guidance is provided by Karaitiana's Tairu and Associates and the Scaffolding Cultural Co-Creativity Project at ANU. And I would say from, you know, this is a cast of thousands, it's not a large project. It's been really interesting, the involvement and support that we've had from so many different glam sector and research groups. And I think it's because of the importance of AI. And I'd like to think as well the approach that we're taking. So it's a small experimental project that aims to understand the potential of large language models and retrieval augmented generation, not retrieval, for transnational historical research. It's purposefully limited in scope to manage rapid technological change. And our North Star is the development of evaluation methods that can lead us to the production of a draft evaluation matrix. And those caveats are important because we're not saying we're going to come up with a final version of this evaluation matrix. In order to reduce the scope, constrain the scope, The sources are limited to Hansard, just in 1901. We chose Hansard because of its political and cultural and historical complexity in terms of interpretation, but also because there are solid sources in the UK, Australia and New Zealand that we can use as the basis of our experiment. And then the stretch goal, which we've actually achieved already thanks to AI Copilot, is the development of a test harness to facilitate manual and automated evaluation of AI research. So we've seen a lot in this conference of point solutions, experiments, prototypes. Our hope is that we can scale that up into a computational harness that can allow us to tweak all the dials and levers and then produce reproducible experiments across a range of sources rather than necessarily just hands-on. So how will we do this? Starting from the bottom up, we want to focus on defining values, culture, and methods first. So one of the great challenges for us is to foreground data sovereignty and indigenous perspectives within the evaluation framework. And that, in many ways, is a design problem and a project management problem, which Karaitiana might be able to speak to next. Once we get the culture values principles in place, Workshops, we've had our first one. Define clear baseline sources so that we can reproduce these experiments using lots of different models, lots of different word embeddings. Have a standard set of prompts as well. So we've got our standard sources, we swap in and out different models. We have prompt databases that we can also reproduce and throw into the different systems. The key of course then is making sure that we track the output and subjectively as well as quantitatively analyze their output. And up the top, point being that once you get those components in place, those test components, you can throw it at lots of different systems and aim for some degree of reproducibility. So what are our emerging questions? I'm going to have to move a bit closer. Fundamentally, how should... Thank you. How should researchers in the GLAM sector judge AI models and judge AI tools? We're at the point where we're experimenting a lot. We haven't even really thought about how we will evaluate these in a structured way and what evaluation vectors, if you will, we'll use to judge these models. It's not just going to be quality of output. It's also presumably going to be alignment to cultural values, environmental impact, cost per prompt. scholarly quality. And what does structured reproducible AI testing look like in the research and GLAM sectors? We can look at the leaderboards, the model leaderboards. They're designed by AI companies to measure their own products. What does an equivalent type of evaluation mean for research and scholarly groups? And I might actually just skip over to Karaitiana now on this bullet point. Can indigenous approaches to knowledge and technology guide us? So our acknowledgement to country at the start of this talk suggests more than just acknowledgement of country. It suggests acknowledgement of technological and cultural know-how that we believe should be woven into the warp and weft of our evaluation of these tools and management of these tools. And that's the reason we're investing in slow AI. We're taking sort of a design first approach to this and foregrounding the problem of how to integrate indigenous evaluation principles into our broader evaluation framework. OK. Should we skip to the slide? The indigenous slide is probably easier. So I guess we're really grateful that under James's leadership that we can bring an indigenous perspective, a true indigenous perspective. But even more better is that this is a trans-Tasman joint indigenous project where we're combining our indigenous knowledges together to create this project. So from a Maori perspective, New Zealand Maori perspective, we're using data sovereignty principles not created by academia, but recognized and created by our elders who also, sorry, I've got to fly around here, who also, yeah, those principles are being recognized in the Waitangi Tribunal in New Zealand. And so they talk about data sovereignty and data being our treasure, like our land. So if we apply the same principles as we would to our land, to our deceased, then we're bringing these principles across. And that includes the care and fear principles. It includes recognising intellectual property rights for both sides of the Tasman for indigenous peoples. The impacts that AI does have on our environment. And so something else that we've realised, and I'm increasingly seeing this over the past several years, consulting with government and the big tech companies in terms of data sovereignty, is tech has grown so much that we kind of need to reevaluate data sovereignty principles, and we need to actually look at indigenous AI principles. So my feeling at this stage, without confirming it, is that I believe that with the current ethical principles, transparent AI, open AI, all the other ethical principles. We could probably just adapt those and then just plug in an indigenous perspective. And then through this project, I'm hoping that we can create a whole brand new indigenous AI and data set of principles. Yeah, and so I guess the big message is to hopefully we can come to the next conference and show you what we've actually achieved. But the work today, this week, and our working group this week, has actually really impressed on me the value of indigenous data sovereignty as an underpinning principle for the evaluation of AI systems. And it's because indigenous data sovereignty principles are founded on embracing complexity, embracing socio-technical and cultural complexity. And we have these draft and actual frameworks that I think can guide us in the way that we interpret the output of these models. but also guide us in the design, engineering, and maintenance of these models. The real key in this project, and I think for all of us, is whether we have enough time to engage genuinely in slow AI. I mean, you've seen just the start of this project. We need six months or a year to come up with anything reasonably robust. and who knows what the technology sector will have come up with in that time. But having said that, I think this is the way to start and we're committed to it and hopefully we'll come back in a year's time and show you something robust and actionable.
The National Film and Sound Archive of Australia acknowledges Australia’s Aboriginal and Torres Strait Islander peoples as the Traditional Custodians of the land on which we work and live and gives respect to their Elders both past and present.