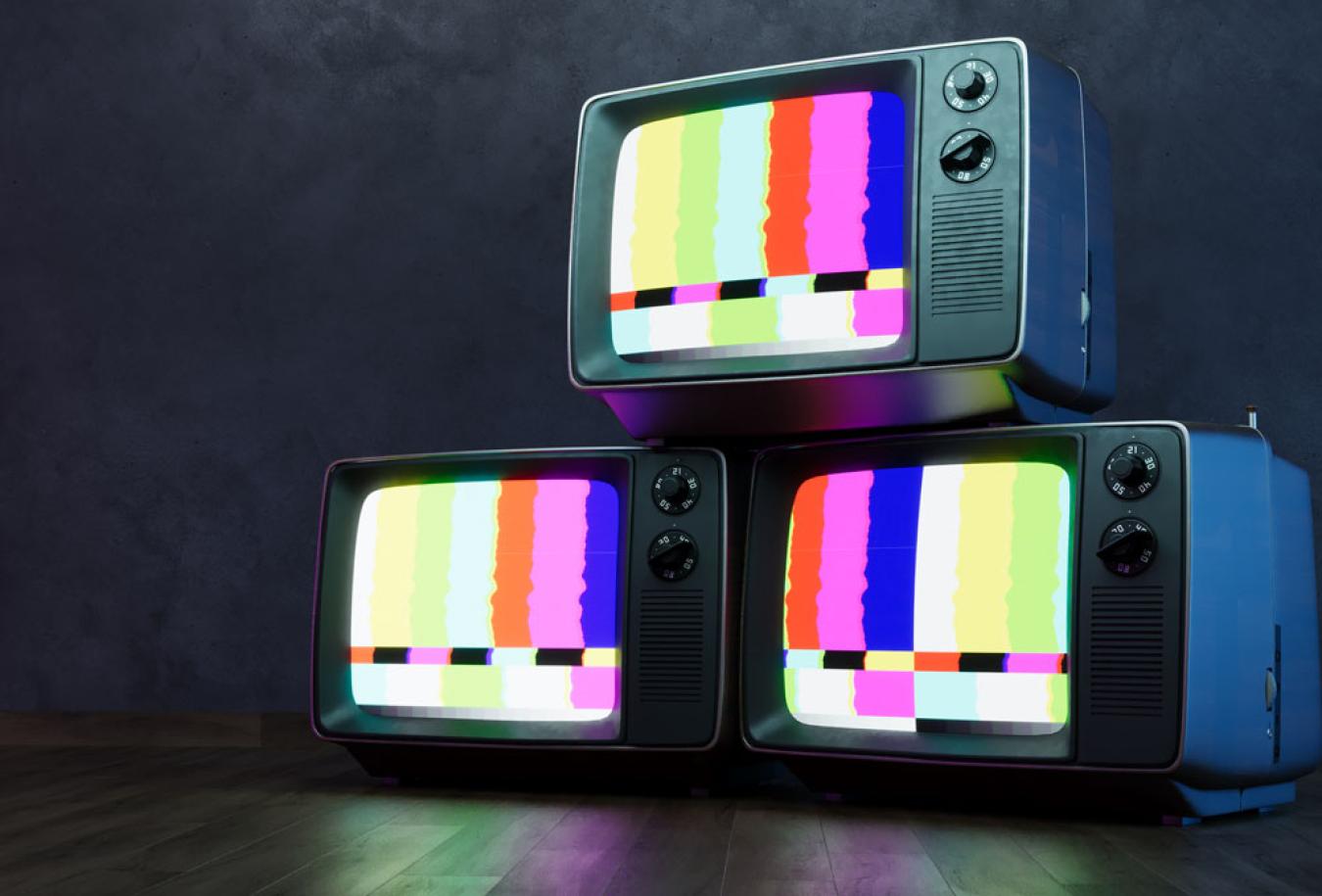
Presenter: Mia Ridge
Ingesting enhanced data appropriately and responsibly into collections management and discovery platforms is a key factor in operationalising AI to improve collections documentation and discovery, but is often difficult in practice. Mia Ridge presents original research into the extent to which projects can integrate enriched data into collections platforms, with success and failure results gathered from over 60 projects.
Technology, language, history and creativity converged in Canberra for four days as cultural leaders gather for the world's first in-depth exploration of the opportunities and challenges of AI for the cultural sector.
Want to learn more about this event?
Visit the Fantastic Futures 2024 Hub
This transcript was generated by NFSA Bowerbird and may contain errors.
Thank you Shahab and I'm glad to know that we're not the, everyone is sort of grappling with these questions of as we generate data what do we do with it, where does it go, how do we ensure that we look after it in the same way that we look after the data that we have generated in a traditional curator, a catalogue, a sitting in front of an item. So just to kind of I'll give you a definition first of enriched metadata. The definition that I use comes from Europeana, which is done, it's a European aggregator. They've done a lot of work to try and augment and supplement the metadata that they get from European collections, because it's often quite sparse, quite poor, particularly when you have collections at scale. So they're just defining it as data about the object that augments or contextualizes or rectifies the authoritative data, which I think is, sometimes it's generous to say that the information produced by a cultural institution is authoritative, sometimes it's rushed, that's fine, it happens. So you might get this enriched metadata through crowdsourcing, through community engagement, asking people to share what they know about your collections. And I think Peter's reminder this morning that tribal objects have names and stories. Cultural organizations have done work at different points, particularly in the mid-2000s. There's a lot of work with working with communities. Asking people to tell the organization what they knew about those collections, what they meant, what the items meant to them. how they operated in the lives of the communities. But we're not quite sure that that data ever really has been looked after well enough that you can find it again. If you did a project in 2006, where is that data now? So it could be created through those kinds of really deep community engagements, talking and meeting with people, or through more micro-task things like correcting, tagging. So obviously Trove is a fantastic example Corrections that you make to OCR newspaper appear straight away. We're not worried about the fact that they're looking after those things. Many other projects, it's not quite clear where that data's gone. And I think particularly when we're asking people to share precious memories, to take their time, to articulate, to trust an institution with their memories and their knowledge, we really need to make sure that we are looking after it in the right way. So I'm coming at this work in machine learning, indexing, topic modeling, whatever, with the knowledge that as organizations, we haven't always been brilliant at using the information. What we're using now is we're using up some of the resources of the planet. We're using energy, water, rare earth minerals to run these machine learning models. So if we're going to generate the data, we should also make sure that we're making the most use of it and retaining it as much as possible. Even when it's not perfect, even when it might be iterated on, we want to tell the story of what we're doing as well. So in part, the research that we did came out of listening to last year's conversations at Fantastic Futures 2023, where a lot of people had thought hard about quality checking, what quality meant, and coming from researching and crowdsourcing, what do you mean by quality is one of the first questions that you ask people who want to do crowdsourcing. How will you know if you have quality data? How will you adjust vocabularies? How will you correct things? do the data transformations. So understanding what data quality means and having those conversations across the organization, what's acceptable, what level of risk in labeling things with computational methods is acceptable. GLAMs are really trusted institutions and they have to think hard about what kinds of information they're putting alongside their collection items online. So maybe terms that we wouldn't use might slip through into language models because they are based on information scraped off the internet and we know what the internet is like. So there's a lot of thinking about how do we integrate this data into our systems, when is it responsible to integrate it into our discovery systems, and how do we make sure that our systems are actually capable of storing this information. So we have lots of debates about quality versus quantity, et cetera. But operationalizing machine learning into the core of what cultural heritage institutions do is very much a kind of work in progress at last year's conference. And I think already we're seeing how far things have come in just a year in terms of systems that are more responsive to what we're trying to do. So we ran a survey. We asked what happens if a project has gathered data enriched by crowdsourcing, machine learning, AI, or a combination of the two. human computation models or correction models. And we asked people, even if you don't work on a project anymore, please feel free to give us a response. Because we know that a lot of earlier projects just kind of finished and didn't finish by ingesting the data. Or maybe people have left a project. So we didn't want it to be only people who are currently in a post. And we also wanted to hear from community groups, Wikipedians, and others who try to work with cultural heritage information but aren't in an institution So we had a very kind of wide invitation and we worked really hard to invite a lot of responses as well, posting and sending individual invitations to do the survey really widely. So we asked what kinds of data people were creating, just to get a sense of like, are we talking about transcriptions, audiovisual, whatever. what kinds of collections management systems people were using. There's been a lot of consolidation in the space, so actually when I get into the results, I can talk about it more, but people are using the same kinds of software mostly these days because there's Axial, there's Alma, there's Ex Libris, and then there's bespoke software. We used to see a huge range of different kinds of software. different collections management systems, but that has changed substantially. We asked about the organizational factors and the technical factors, data quality, whether middleware was in play, because most organizations need some kind of glue to manage links between their different systems, their digital asset management system, perhaps, and their collections management system. And we also asked optional questions about the organization and the person. So, really quickly, we heard mostly from libraries. And I think that kind of speaks partly to who the AI for LAM community is. It's quite library focused. I work in a library now having worked in museums. Maybe librarians are just chattier. But I think also because libraries have done so many of these projects and I think in the museum sector there's been a lot more churn in terms of people who work in technology who maybe don't have longer histories or roots in the field and aren't as connected to the sector as people used to be perhaps. And also a lot of institutions are multi-purpose. So we heard a lot of university libraries, archives, galleries, et cetera, but mostly libraries. So I'd love to do another version of this and in particular translate the survey so that we can hear back from people who aren't comfortable answering questions in English, but also to understand whether the questions that we're asking translate into different contexts. So there is a kind European, North American preponderance and UK as well in terms of who we've reached. So, yeah, another time I'd love to reach wider groups and smaller organizations as well. So maybe working with national institutions to reach the kind of membership organizations. And most projects were crowdsourcing. But quite a few were a combination of crowdsourcing and AI or machine learning in some way and some were machine learning without any form of like crowdsourcing or public participation in the project. And I should say when I talk about crowdsourcing, I know Peter mentioned crowdsourcing this morning, that was very much a kind of mechanical Turk model where you are paying people and you're sort of extracting knowledge from their brains. The kind of crowdsourcing that we're talking about in cultural heritage organisations is much more part of a kind of movement towards public engagement, public digital engagement, getting people to work with institutions to work together and to share what we're doing as we go. So it's, the idea is always that the people who are volunteering their time get as many benefits from the process as they do, as the institutions do. So overall we found that actually most people were able to ingest data, either new records or updated records, and that was one of the key things that we were asking is like, can you just wholesale import new records or can you manage a slightly trickier process of dealing with mergers and replication issues, so, you know, Do you have ID systems in place so that you can update and target the right part of the right record that you want to update? So I was actually really encouraged by this in terms of I expected a lot more people to say that they weren't able to do things. And I think that partly speaks to the maturation of the field where we're having wider all organization conversations. Even things like chat GPT in terms of making everyone think, what do we need to be thinking about with AI? And I think also the sharing that people do at events like this, on mailing lists, on slacks, on social media in the form that it still exists, is really important for helping people understand how to translate this work into their local context and how to learn from each other, share workflows, share methods, share tips and tools. The conversations that you're having here and that you hopefully go back and have in your institutions and online are really important. So to look at some of the factors in success. And when I get onto the barriers, a lot of the barriers are the flip side of this success. So if you don't do these things, then that can be one of the reasons why projects didn't succeed. And it was really striking in reading through the responses, and I've done a word cloud because I'm being retro. Um, is that a lot of the conversations, a lot of the responses from people who were successful began with the word agreement, or they talked about collaboration. Um, so talking like voting, do it early and often. Um, it's a really important factor where otherwise in the barriers issue, um, people would just sort of hand over a bunch of data and expect that someone else was going to drop everything, figure out what they had, figure out whether it was authoritative, if it needed quality control. and like slurp it into their systems, that's not going to happen. You're not going to go, thanks for the USB drive of random stuff. You need to have these conversations. You need to understand what you're asking of other departments, other organizations, other teams, and discuss things like specifications, standards, like all the nerd-tastic stuff that actually makes these projects work. You can't get anywhere without talking to people. So things like, you know, are you asking where is the burden? Who is going to agree to take on these responsibilities? And the other kind of really key factor was infrastructure that responds to needs. Some organizations make their own bespoke software. Some have made smart decisions about software that supports APIs, that supports imports and exports, that uses standard formats. They've had conversations about controlled vocabularies, where they're necessary, where they're not necessary. Thinking about the pipelines, some people have entirely manual processes, and that's fine, because they've made them replicable, so they can repeat them again and again, even if there is manual work involved. And the great thing about smart workflows is if you can take out a bit and automate some of it, then the whole thing improves. So thinking about workflows, again, really boring, but actually really vital to making the most of our investment in machine learning and AI. So that's really important, but some of the barriers include lack of technical skills. So someone's done a project and their institution doesn't have anyone technical on staff or people leave. Not anti-Mark. Mark is a library standard, but it is line noise. And it doesn't really respond to the need that we have for generous thinking. I don't know if Mitchell's here, but generous interfaces, generous thinking about the kinds of information we can store in our cataloging and discovery systems. We don't have to squeeze it all into dollar signs. Our inability to ingest third party metadata, we don't have fields to store things, we don't have the ability to attach files, we don't have those middleware systems that let you say here are additional bits of information about this record. Gatekeeping and institutional politics, everybody's favorite topic, can be a huge barrier. And again, flip side, talk to people, understand their concerns, understand the issues that they're facing, and try and work to resolve them together. Lack of time, probably very familiar to all of us. And sometimes it's just that a project didn't produce data that was of a high enough quality. And then things like you don't have IDs, so you can't match things between systems easily. A lot of these can be solved eventually with automatic methods, but for conversations, computers can't do that bit for you. And finally, there are some alternatives to ingesting data, like did you ingest data is not the only marker of success. Sometimes it can be that you can make your changes directly into the system. So in Trove, you make a correction to OCR and it appears. If you make an edit to a wiki, it appears. Some people sidestep the core systems, so they might have like a microsite, again, being a bit retro. Or they might have an entirely standalone interface designed specifically around the data for that collection. Or you might just do nothing with it. And I hope you don't choose that last option, but that you find ways to take all the work that you're doing and make sure that it's in your systems in a responsible way for the long term. So thank you for listening. And we're not having questions, but come and chat to me if you have any.
The National Film and Sound Archive of Australia acknowledges Australia’s Aboriginal and Torres Strait Islander peoples as the Traditional Custodians of the land on which we work and live and gives respect to their Elders both past and present.